Empowering Retail Growth with Scalable Cloud Infrastructure
Consumer demands are rapidly changing, forcing retailers to adapt their supply chain, inventory management, and customer engagement strategies to remain competitive. As expectations shift, the importance of cloud infrastructure in driving retail growth becomes more pronounced. Cloud technology offers the scalability and agility needed to address fluctuating customer demands, particularly during peak seasons and promotional events. By leveraging cloud solutions, retailers can optimize operations and enhance the overall customer experience. This adaptability is essential for sustainable growth in a dynamic retail landscape.
In today’s digital-first environment, consumers expect convenience, personalization, and seamless experiences across both online and physical channels. Cloud infrastructure helps retailers meet these demands by centralizing data and streamlining processes by utilizing data analytics, artificial intelligence, and machine learning and gains valuable insights into consumer behavior and preferences. This knowledge allows for refined marketing strategies and optimized inventory management. Embracing cloud technology enables retailers to navigate the evolving retail sector with agility and efficiency, positioning them for long-term success.
Role of Cloud Infrastructure in Retail Transformation
Cloud infrastructure is transforming the retail industry by offering unparalleled scalability, especially during peak shopping seasons. Thanks to the elasticity of cloud computing, retailers can dynamically adjust computing resources to handle spikes in web traffic and transactions effortlessly. With features like cloud-based load balancing and content delivery networks (CDNs), retailers can optimize performance and minimize latency during high-demand periods. Additionally, autoscaling capabilities allow retailers to automatically scale resources, ensuring seamless operations during peak times and enhancing the customer experience.
Moreover, cloud infrastructure provides robust data management solutions essential for modern retail. Retailers can securely store and analyze vast amounts of customer data, sales transactions, and inventory details, gaining key insights into consumer behavior, preferences, and market trends. This data-driven approach enables personalized marketing strategies and optimized product offerings, driving customer loyalty and business growth. By leveraging cloud-based data management, retailers can run targeted campaigns, predict demand, and improve the customer experience across multiple channels, strengthening their competitive edge in the retail market.
Meeting Growing Retail Demands Seamlessly
Scalable cloud solutions are essential for retailers to manage fluctuating customer demands, especially during peak seasons. With cloud infrastructure, retailers can dynamically adjust their operations to handle varying levels of demand, ensuring consistent performance and high customer satisfaction during high-traffic periods. Cloud-based load balancing and content delivery networks (CDNs) allow retailers to efficiently manage web traffic, reduce latency, and enhance the shopping experience.
Additionally, cloud technology empowers retailers to leverage advanced data management and analytics for informed decision-making based on market trends and consumer behavior. By securely storing and analyzing large volumes of customer data, retailers can refine marketing strategies, predict demand, and optimize product offerings to align with changing customer preferences. Cloud-based solutions seamlessly integrate big data and analytics, enabling targeted campaigns and enhancing the customer experience across multiple channels, demonstrating the agility and customer-centric approach necessary to meet the evolving demands of the retail industry.
Maximizing Retail Efficiency with Cloud Infrastructure
Heightened data security: Cloud infrastructure provides robust data security measures, including encryption, access controls, and regular backups, safeguarding sensitive customer information and business data from potential threats.
Enhanced user experience: By leveraging cloud technology, retailers can deliver seamless and personalized experiences across multiple touchpoints, ensuring a cohesive and responsive interaction with customers, and ultimately fostering brand loyalty.
Improved inventory management: Cloud-based inventory systems enable real-time tracking, demand forecasting, and automated replenishment, optimizing stock levels, reducing holding costs, and minimizing stockouts, thereby improving operational efficiency.
Effective disaster management: Cloud infrastructure offers data redundancy, off-site backups, and disaster recovery capabilities, ensuring minimal disruptions and swift recovery in the event of unforeseen incidents such as system failures or natural disasters.
Increased profitability: The agility and scalability of cloud solutions support cost-efficient operations, enabling retailers to respond swiftly to market demands, reduce infrastructure costs, and allocate resources effectively, ultimately contributing to increased profitability and sustainability.
Cloud Shapes Future Retail Growth Landscape
In the future, cloud technology is set to revolutionize retail, driving efficiency and personalized experiences. Retailers will increasingly rely on cloud-based solutions for real-time data analytics and operational agility across digital and physical channels. The integration of AI, machine learning, and IoT with cloud computing will enhance engagement and enable retailers to adapt to changing market dynamics.
Cloud technology’s future in retail growth also promises improved resilience and adaptability, empowering retailers to swiftly respond to market shifts and disruptions. This flexibility will enable retailers to thrive in a competitive digital landscape by enhancing operational agility and driving growth through data-driven strategies.
AI-Driven Data Analytics: Transforming Politics and Securing Elections
Artificial intelligence is transforming the political landscape, driving more sophisticated campaigns and enhancing election security. With the capabilities of AI-driven data analytics, political teams can now sift through massive datasets to identify trends, understand voter behavior, and create targeted messaging that truly resonates with voters. This ability to adapt in real-time ensures that campaigns remain relevant and impactful, tailoring their approaches to the ever-changing political environment.
Beyond shaping campaign strategies, AI also plays a crucial role in safeguarding election integrity. By detecting anomalies in voter data and identifying potential fraud, AI helps maintain the transparency and security of the electoral process. As concerns about election security and transparency continue to grow, AI becomes an indispensable tool in protecting the democratic process and ensuring fair and credible elections.
AI-Enhanced Voter Behavior Insights: Data-Driven Political Decisions
Imagine a world where political messages seem to speak directly to interests and concerns—that’s the transformative power of AI-driven analytics in action. Voters are experiencing a new level of engagement and relevance in political content, thanks to advanced AI tools like Crimson Hexagon and PoliMonitor that analyze vast datasets, including voting history, social media activity, and demographic information. These AI systems offer campaigns deep insights into voter preferences, enabling them to craft highly personalized messages that resonate on an individual level.
This tailored approach means that voters receive content that truly addresses their specific needs and concerns, rather than generic, one-size-fits-all messaging. Additionally, AI’s ability to predict and track changes in voter sentiment in real-time allows campaigns to adjust their strategies dynamically. This responsiveness ensures that political communication remains timely and effective, adapting to shifting attitudes and emerging issues. As AI technology continues to advance, its impact on political messaging is set to deepen, making interactions with campaigns more relevant and engaging, and ensuring that voters are better informed and more connected to the issues that matter most to them.
Detecting and Preventing Election Fraud with AI Algorithms
AI algorithms are revolutionizing the way election fraud is detected, ensuring greater transparency in democratic processes. By analyzing large datasets, AI can swiftly identify irregularities such as duplicate registrations and unusual voting patterns, significantly reducing the potential for human error. Governments are increasingly adopting these technologies to safeguard election integrity, making AI an essential tool in fraud prevention.
Moreover, AI goes beyond mere detection by utilizing predictive analytics to anticipate potential risks and monitoring social media to combat misinformation. As elections become more digitized, AI’s role in maintaining security and fairness is becoming increasingly critical. This underscores the importance of AI for government agencies tasked with election security, as it helps protect against cyber threats and ensures the integrity of election data and processes.
Predictive Analytics: Shaping Campaign Strategies and Election Outcomes
Predictive analytics is transforming campaign strategies and election outcomes by harnessing AI to analyze voter data and trends. By examining information from social media, voting history, and demographics, AI enables campaigns to forecast voter behavior with remarkable accuracy. This capability allows political teams to craft highly targeted messages and strategies tailored to specific voter segments, optimizing their outreach and engagement efforts.
Additionally, AI-powered predictive analytics enhances campaign efficiency by facilitating real-time adjustments. It helps campaigns anticipate shifts in voter sentiment and opponent strategies, enabling better resource allocation and more effective voter engagement. As AI technology continues to advance, its influence on shaping election strategies and outcomes is expected to grow, offering deeper insights and more precise tools for both political analysts and campaign teams.
Social Media Sentiment Analysis: AI’s Role in Political Messaging
AI-driven social media sentiment analysis is transforming political analysis by offering deep insights into voter behavior and election trends. For political analysts, AI tools provide real-time data on public sentiment, enabling more accurate predictions and strategic advice. This enhanced analysis helps campaigns refine their messaging and engage more effectively with voters.
For voters, AI ensures that political content is relevant and engaging, improving the quality of information and interaction. Additionally, government agencies use AI to protect election integrity and combat cyber threats, making elections more secure. As AI technology advances, its impact on political messaging and election security continues to grow.
AI in Election Auditing: Enhancing Transparency and Security
AI in Election Auditing is revolutionizing the way election committees ensure transparency and security. By utilizing advanced AI tools, these committees can efficiently monitor and audit election processes, quickly identifying irregularities and potential fraud. This technology enhances transparency by detecting discrepancies and maintaining trust in the electoral system.
Political analysts also gain from AI, using it to delve deeper into voter behavior, election trends, and public sentiment. AI provides critical insights that improve predictions and strategic advice, leading to more informed and effective election strategies. As AI continues to advance, its impact on both auditing and analyzing elections is becoming increasingly vital for ensuring a secure and transparent democratic process.
Ethical Challenges and the Future of AI in Politics and Election Security
As AI advances in politics and election security, it presents both significant benefits and ethical dilemmas. While AI enhances voter targeting, sentiment analysis, and election integrity, it also raises concerns about privacy, data manipulation, and misuse. These issues underscore the need for strict ethical guidelines to protect voter information and ensure fair practices.
The future of AI in politics will depend on addressing these ethical challenges while leveraging its potential. Ensuring transparency, data protection, and clear regulations will be key to maintaining trust and fairness in elections. Balancing innovation with ethical considerations is essential for upholding democratic values as AI technologies continue to evolve.
Hybrid Cloud Revolution: A Modern IT Infrastructure Solution
Hybrid cloud combines on-premises infrastructure with public cloud services, like AWS, to create a flexible, scalable IT environment. It allows businesses to run workloads locally and in the cloud, optimizing performance, security, and compliance. This architecture offers the agility to manage data where it’s most efficient, balancing the benefits of cloud innovation with the control of in-house systems.
With AWS hybrid cloud solutions such as AWS Outposts and AWS Direct Connect, businesses can seamlessly integrate their existing infrastructure with the cloud. This enables efficient scaling, low-latency connections, and adherence to regulatory standards, providing the best of both on-premises and cloud environments.
Why do businesses implement a hybrid cloud?
Businesses adopt hybrid cloud solutions to integrate their on-premises infrastructure with cloud services like AWS, enhancing control and flexibility. This approach allows for smoother migration and modernization by combining existing IT systems with AWS’s scalability and cost-efficiency. Companies can keep sensitive workloads on-premises while utilizing AWS for its powerful tools and resources, which help meet low-latency needs, process data locally, and comply with regulations.
AWS plays a crucial role in hybrid cloud strategies by offering scalable resources that can adjust to varying demands, reducing hardware investments and operational costs. Additionally, hybrid cloud setups ensure business continuity by enabling workload shifts between on-premises and cloud environments, minimizing disruptions during maintenance or failures. This blend of local and cloud resources helps businesses maintain a resilient and adaptable IT infrastructure.
Key Benefits of Hybrid Cloud for Modern Businesses
Hybrid cloud infrastructure provides modern businesses with a powerful blend of on-premises and cloud solutions, leveraging the best of both worlds to enhance operational efficiency and flexibility. One of the primary advantages of a hybrid cloud is increased scalability. By integrating AWS cloud services with existing on-premises resources, companies can dynamically scale their compute and storage capabilities based on demand. This elasticity ensures that businesses can handle varying workloads efficiently without over-provisioning resources, thereby optimizing costs and performance.
Another significant benefit is improved agility in development and deployment. With a hybrid cloud setup, businesses can leverage AWS’s extensive suite of services to accelerate application development, testing, and deployment. This approach allows for rapid experimentation and innovation, as developers can utilize AWS’s scalable infrastructure to test new ideas and roll out solutions faster than traditional setups. The ability to seamlessly integrate and manage these services across environments further supports continuous integration and delivery practices.
Hybrid cloud solutions also bolster business continuity and resilience. In scenarios where on-premises systems are undergoing maintenance or facing issues, AWS’s public cloud can take over, ensuring minimal disruption to operations. This capability is crucial for maintaining high availability and reducing downtime, which directly impacts customer satisfaction and operational efficiency.
Lastly, hybrid cloud environments enhance compliance and data security. By using AWS’s secure cloud services in conjunction with local data centers, businesses can ensure that sensitive data is stored and managed according to regulatory requirements. This hybrid approach provides greater control over data residency and security policies, helping businesses meet stringent compliance standards while taking advantage of cloud innovation.
Hybrid Cloud with AWS: A Strategic Approach to Modern IT Infrastructure
In today’s rapidly evolving digital landscape, many organizations are turning to hybrid cloud solutions to achieve greater flexibility and scalability. AWS, with its comprehensive suite of cloud services, stands out as a leader in this space. The hybrid cloud strategy offered by AWS allows businesses to seamlessly integrate on-premises infrastructure with AWS’s robust cloud environment. This approach enables organizations to leverage the scalability and cost-efficiency of AWS while maintaining control over sensitive data and legacy systems on-premises. Key AWS services like AWS Direct Connect and AWS Storage Gateway facilitate this seamless integration, ensuring a smooth and secure connection between local data centers and the cloud.
AWS’s hybrid cloud strategy is designed to address the diverse needs of businesses looking to optimize their IT operations. By utilizing services such as Amazon EC2, which provides scalable compute capacity, and Amazon S3, which offers secure and scalable storage, companies can build a hybrid environment that supports a wide range of applications and workloads. This strategic blend of on-premises and cloud resources allows organizations to achieve enhanced performance, agility, and cost-effectiveness. As businesses continue to navigate the complexities of modern IT infrastructure, AWS’s hybrid cloud solutions offer a compelling path forward, combining the best of both worlds to drive innovation and operational excellence.
Building a Hybrid Cloud Strategy for Future Growth
In today’s rapidly evolving technological landscape, adopting a hybrid cloud strategy is becoming increasingly crucial for businesses aiming to scale and innovate. A hybrid cloud environment, which integrates both on-premises infrastructure and public cloud services, offers unparalleled flexibility and agility. By leveraging platforms like AWS, organizations can seamlessly manage workloads across different environments, ensuring optimal performance and cost-efficiency. AWS’s extensive suite of cloud services, including AWS Lambda and Amazon EC2, provides businesses with the tools they need to dynamically allocate resources and adapt to changing demands.
Implementing a hybrid cloud strategy with AWS not only enhances operational efficiency but also fortifies data security and compliance. AWS’s robust security features, such as AWS Shield and AWS Key Management Service (KMS), ensure that sensitive information is safeguarded across both on-premises and cloud environments. Furthermore, the integration of AWS’s cloud-native tools with existing on-premises infrastructure enables organizations to harness the full potential of cloud computing while maintaining control over their data and applications. This strategic approach to cloud adoption empowers businesses to future-proof their IT infrastructure, drive innovation, and support sustained growth in an increasingly competitive market.
Being an AWS Select Partner offers a range of advantages for organizations focusing on hybrid cloud solutions. This partnership provides exclusive access to advanced training and technical support, ensuring that partners are well-equipped to implement and optimize AWS hybrid cloud strategies. AWS Select Partners also benefit from the AWS Partner Network (APN) Portal, which includes specialized tools and resources to enhance their hybrid cloud offerings. Moreover, the partnership opens doors to co-marketing opportunities and collaborative go-to-market initiatives, empowering partners to showcase their expertise and expand their reach in delivering innovative hybrid cloud solutions with AWS.
Fashion Forward: The Generative AI Revolution in E-commerce Retail
As the e-commerce industry evolves, retailers face new challenges and opportunities, making it crucial to integrate Generative AI into their business strategies. This transformative technology is revolutionizing both front-end customer interactions and back-end processes, from personalized search suggestions and AI-generated collections to localized product content. By embracing Generative AI, brands can offer highly personalized shopping experiences, enhance customer engagement, and drive innovation, ensuring they stay competitive in a rapidly changing market. In this blog, we delve into the profound impact of Generative AI on e-commerce, exploring its role in personalized shopping, virtual try-ons, predictive fashion trends, and the future of AI-powered business models.
Personalized Shopping Experiences: AI in eCommerce
Personalization has become the cornerstone of modern eCommerce, and AI is at the forefront of delivering this tailored shopping experience. Generative AI analyzes vast amounts of customer data, including browsing history, past purchases, and even social media behavior, to create individualized recommendations. These personalized suggestions ensure that customers are presented with products that align with their preferences, increasing the likelihood of a purchase.
Beyond product recommendations, AI is enhancing the overall shopping journey. AI-driven chatbots provide real-time assistance, answering customer queries and guiding them through the buying process. These virtual assistants are not just reactive; they proactively suggest products, offer discounts and provide personalized content based on the customer’s behavior. This level of customization not only improves the customer experience but also fosters brand loyalty, as shoppers feel that the brand understands and caters to their unique needs.
Moreover, visual search capabilities powered by AI allow customers to upload images and find similar products, revolutionizing the way people discover new items. This feature is particularly valuable in the fashion industry, where style and aesthetics play a crucial role in purchase decisions. By leveraging AI, eCommerce platforms are creating a more intuitive, engaging, and personalized shopping experience that resonates with today’s consumers.
Virtual Try-Ons: Redefining Customer Engagement
One of the most significant challenges in online fashion retail is the inability for customers to physically try on products before making a purchase. Generative AI is addressing this issue through virtual try-ons, a technology that allows customers to see how clothes, accessories, or makeup would look on them without leaving their homes.
Virtual try-ons use AI to analyze a customer’s body shape, skin tone, and facial features, creating a realistic representation of how products will fit and appear. This technology not only enhances customer confidence in their purchase decisions but also reduces return rates, as customers are more likely to choose items that suit them well.
For example, AI-powered virtual fitting rooms enable shoppers to mix and match outfits, experiment with different styles, and see the results in real time. This interactive experience bridges the gap between online and in-store shopping, offering the convenience of eCommerce with the assurance of a fitting room experience. By redefining customer engagement through virtual try-ons, AI is helping retailers create a more immersive and satisfying shopping experience.
Predictive Fashion Trends: How AI is Shaping the Future
The fashion industry is notoriously fast-paced, with trends emerging and fading at a rapid pace. Generative AI is playing a pivotal role in predicting these trends, enabling retailers to stay ahead of the curve. By analyzing data from various sources, including social media, fashion shows, and consumer behavior, AI can identify emerging trends before they become mainstream.
This predictive capability allows retailers to optimize their inventory, ensuring they stock the right products at the right time. For instance, AI can forecast the popularity of certain styles, colors, or materials, enabling brands to respond quickly to changing consumer preferences. This agility is crucial in the fashion industry, where timing is everything.
Moreover, AI can help designers and brands experiment with new ideas, generating innovative designs based on current trends. These AI-generated designs can inspire fashion lines, allowing brands to create unique collections that resonate with consumers. By leveraging AI’s predictive power, retailers can not only keep up with the latest trends but also set new ones, establishing themselves as industry leaders.
How AI is Revolutionizing the Retail Industry
The impact of AI on the retail industry extends beyond eCommerce and fashion. Across the entire retail landscape, AI is driving innovation, efficiency, and customer satisfaction. From supply chain optimization to in-store experiences, AI is revolutionizing how retailers operate and interact with customers.
In supply chain management, AI is improving efficiency by predicting demand, optimizing inventory levels, and reducing waste. Machine learning algorithms analyze sales data, seasonal trends, and external factors such as economic conditions to forecast demand accurately. This enables retailers to manage their inventory more effectively, ensuring that popular products are always in stock while minimizing excess inventory.
In physical stores, AI is enhancing the shopping experience through technologies such as smart mirrors, automated checkout systems, and personalized promotions. Smart mirrors, for instance, allow customers to try on clothes virtually, offering styling suggestions based on their preferences. Automated checkout systems use AI to streamline the payment process, reducing wait times and improving customer satisfaction.
Moreover, AI-driven personalization extends to in-store promotions, where customers receive tailored offers based on their purchase history and behavior. This level of customization ensures that promotions are relevant, increasing the likelihood of a sale and improving the overall shopping experience.
The Future of Retail: AI-Powered Business Models
As AI continues to evolve, it is paving the way for new business models in the retail industry. AI-powered platforms are enabling retailers to offer highly customized products and services, catering to the specific needs and preferences of individual customers.
One emerging business model is the concept of hyper-personalization, where AI tailors every aspect of the shopping experience to the individual customer. This goes beyond product recommendations and extends to personalized pricing, marketing, and even product design. By leveraging AI, retailers can create unique experiences for each customer, differentiating themselves in a competitive market.
Another promising development is the rise of AI-driven marketplaces, where algorithms match customers with products and services that best meet their needs. These platforms use AI to analyze customer data, predict preferences, and curate personalized shopping experiences. This not only enhances customer satisfaction but also allows smaller brands to reach their target audience more effectively.
Generative AI is transforming the e-commerce retail industry by seamlessly integrating online and offline experiences, ensuring customers receive personalized and consistent interactions across all channels. By tracking online behavior, AI tailors in-store experiences with customized recommendations and promotions, enhancing customer engagement and satisfaction. As AI continues to advance, it is not only driving innovation in personalized shopping and virtual try-ons but also predicting fashion trends and unlocking new business models. Retailers who embrace this technology will be at the forefront of a fashion-forward, customer-centric future in commerce.
Supercharging AWS Cloud Operations: A Journey from Docker to Kubernetes
Understanding the Docker-Kubernetes-AWS Ecosystem
The Docker-Kubernetes-AWS ecosystem forms a robust foundation for modern cloud operations. Docker streamlines the packaging and deployment of applications by containerizing them, ensuring consistent environments across different stages of development. Kubernetes, an orchestration tool, takes this a step further by automating the deployment, scaling, and management of these containers, providing resilience and high availability through features like self-healing and load balancing.
Integrating this with AWS amplifies the ecosystem’s capabilities. AWS offers scalable infrastructure and managed services that complement Kubernetes’ automation, like Amazon EKS (Elastic Kubernetes Service), which simplifies Kubernetes deployment and management. This trio enables organizations to build, deploy, and manage applications more efficiently, leveraging Docker’s portability, Kubernetes’ orchestration, and AWS’s extensive cloud infrastructure. Together, they create a seamless, scalable, and resilient environment that is crucial for cloud-native applications.
Why is Kubernetes the Next Step After Docker on AWS?
After mastering Docker on AWS, Kubernetes emerged as the natural progression for scaling containerized applications. While Docker simplifies container management, Kubernetes takes it further by orchestrating these containers at scale, providing advanced features like automated scaling, self-healing, and seamless rollouts. This level of automation is essential for handling complex workloads in a dynamic cloud environment like AWS, where demands can shift rapidly.
Kubernetes also offers a significant advantage over Docker Swarm in terms of flexibility and resilience. With Kubernetes, you can deploy multi-region, multi-cloud applications effortlessly, ensuring high availability and fault tolerance. Its robust ecosystem, including Helm for package management and integrated CI/CD pipelines, streamlines cloud operations, reducing manual interventions and minimizing downtime. In an AWS environment, Kubernetes leverages services like Amazon EKS, enabling you to manage clusters with AWS-native tools, which enhances security, compliance, and cost efficiency.
In summary, Kubernetes on AWS empowers cloud operations teams with unparalleled control, scalability, and efficiency, making it the logical next step after Docker for enterprises aiming to optimize their cloud-native architectures.
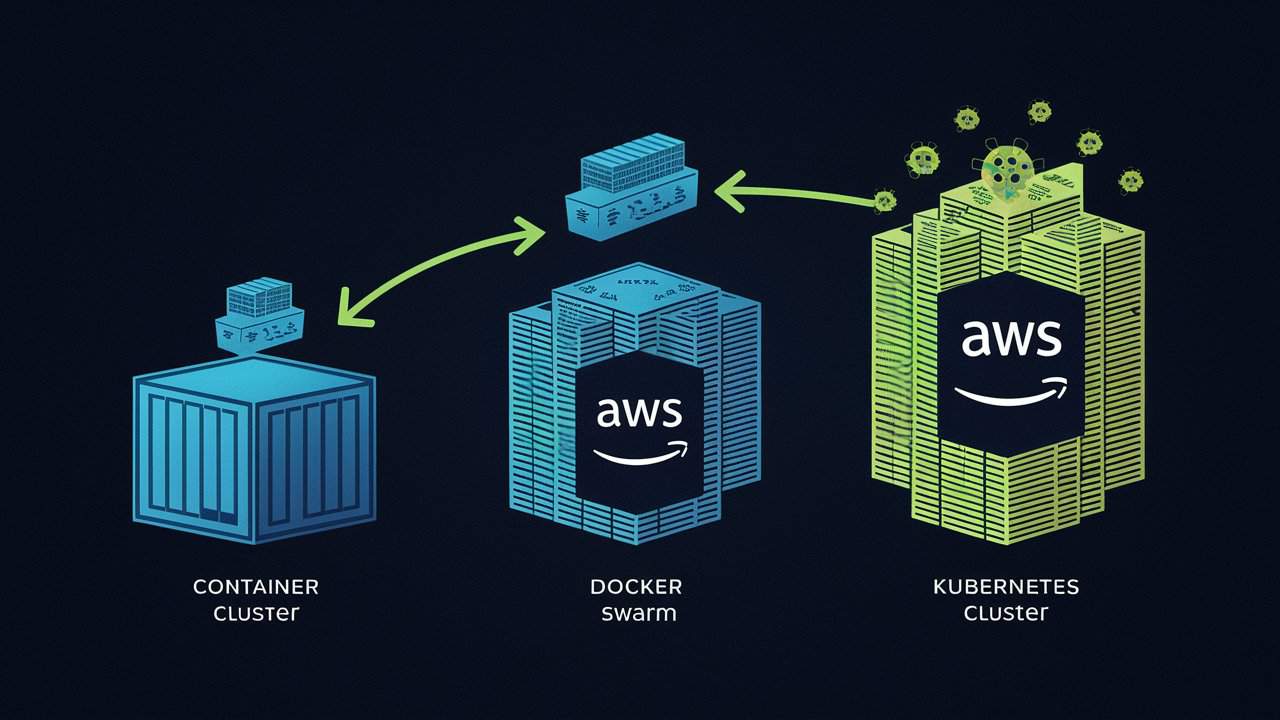
Container Orchestration Simplified: How Kubernetes Enhances Docker Workloads
Container orchestration is pivotal for scaling and managing containerized applications, especially in cloud environments. Kubernetes, an open-source platform, simplifies this by automating the deployment, scaling, and management of Docker containers. It acts as a robust control plane, ensuring that containerized applications run seamlessly across clusters, regardless of underlying infrastructure.
In cloud operations, Kubernetes enhances Docker workloads by providing resilience, scalability, and efficient resource utilization. It automatically handles load balancing, self-healing, and rolling updates, ensuring minimal downtime and consistent application performance. By abstracting the complexity of managing multiple containers, Kubernetes allows cloud teams to focus on application development rather than infrastructure management.
Moreover, Kubernetes integrates smoothly with cloud services like AWS, GCP, and Azure, offering built-in tools for monitoring, logging, and security. This integration ensures that cloud-native applications can scale effortlessly, adapt to changing demands, and maintain high availability, making Kubernetes an indispensable tool for modern cloud operations.
Efficient Application Scaling for Cloud Developers with Docker, Kubernetes, and AWS
Efficient application scaling is vital for cloud developers, and integrating Docker, Kubernetes, and AWS offers a robust solution. Docker streamlines application deployment by encapsulating it in lightweight containers, ensuring consistent performance across different environments. These containers can be easily scaled up or down based on real-time demand, simplifying the management of varying workloads.
Kubernetes enhances this process by orchestrating these containers at scale. As an open-source platform, Kubernetes automates deployment, scaling, and operational management of containerized applications, allowing developers to concentrate on development rather than infrastructure. When paired with AWS, Kubernetes benefits from the cloud provider’s comprehensive ecosystem, including Elastic Kubernetes Service (EKS), which facilitates seamless cluster management.
AWS further supports scalable cloud operations with Auto Scaling and Elastic Load Balancing. These services automatically adjust resources to match traffic fluctuations, maintaining application responsiveness and optimizing cost efficiency. Together, Docker, Kubernetes, and AWS provide a cohesive framework for efficient, scalable cloud management.
Streamlined CI/CD Pipelines: Leveraging Kubernetes in Your Docker-Based AWS Environment
Streamlined CI/CD pipelines are essential for optimizing cloud operations, particularly when integrating Kubernetes with Docker in an AWS environment. Kubernetes automates the deployment, scaling, and management of Docker containers, making it easier to manage complex applications. This orchestration simplifies updates, enhances rollback capabilities, and minimizes downtime, ultimately boosting operational efficiency.
In AWS, combining Kubernetes with Docker leverages the full power of scalable, resilient infrastructure. Services like Amazon EKS (Elastic Kubernetes Service) manage Kubernetes clusters, allowing you to focus on application development rather than infrastructure maintenance. This integration fosters a more agile development process, accelerating time-to-market while ensuring high availability and performance. By aligning your CI/CD practices with these technologies, you achieve a more efficient and reliable cloud operation, meeting the demands of modern software delivery.
Optimizing Docker Swarm and Kubernetes on AWS: Key Takeaways
Optimizing Docker Swarm and Kubernetes on AWS can significantly enhance cloud operations, leading to more efficient resource utilization and streamlined deployments. Docker Swarm’s simplicity is a strong advantage for managing containerized applications. Leveraging AWS features such as Elastic Load Balancing (ELB) and Auto Scaling with Docker Swarm can ensure high availability and dynamic scaling. Utilizing AWS Fargate with Docker Swarm can further optimize operations by removing the need for managing underlying infrastructure, thereby reducing overhead and simplifying management.
On the other hand, Kubernetes provides more advanced orchestration capabilities and is ideal for complex, microservices-based applications. AWS EKS (Elastic Kubernetes Service) integrates seamlessly with Kubernetes, offering managed control plane operations, which simplifies cluster management and scales effortlessly with demand. To optimize Kubernetes on AWS, leverage AWS CloudWatch for monitoring and AWS IAM for fine-grained security controls. Combining Kubernetes’ robust orchestration with AWS’s scalable infrastructure ensures resilient, cost-effective, and highly available cloud operations.
Precision and Progress: Advancing Manufacturing with Generative AI
The manufacturing industry has evolved from manual craftsmanship in ancient times to the mechanized production of the Industrial Revolution, and further to the automation era of the 20th century. Despite these advancements, the industry has long faced complaints about inefficiencies, waste, high costs, and inconsistent quality. Workers also raised concerns about unsafe conditions and job displacement due to automation. The constant pursuit of innovation has driven the industry to seek solutions, culminating in today’s adoption of advanced technologies like AI.
Today, Generative AI is at the forefront of this evolution, bringing transformative changes to the industry. By leveraging algorithms that can generate designs, optimize processes, and predict outcomes, generative AI is revolutionizing how products are conceived and produced. In manufacturing, it enables the creation of complex, innovative designs that were previously impossible, while also streamlining production workflows. From reducing material waste to enhancing product quality, generative AI is not just a tool but a game-changer, driving the next wave of innovation and competitiveness in the manufacturing sector. The future of manufacturing is being shaped by these intelligent, adaptive technologies.
Enhancing Design Accuracy with AI-Driven Modeling
AI-driven modeling is revolutionizing design accuracy in the manufacturing industry, setting new standards that surpass traditional methods. Conventional design processes often involve extensive trial and error, which can be time-consuming and costly. In contrast, generative AI algorithms analyze vast datasets to create precise models, optimizing for factors such as material efficiency, durability, and cost. These algorithms can simulate thousands of design variations, ensuring that the final product is not only innovative but also meets exact specifications. This high level of precision reduces errors and minimizes the need for costly revisions, resulting in products that perform reliably in real-world conditions.
Moreover, AI-driven modeling enables rapid prototyping and testing, significantly speeding up the design process. Engineers can quickly visualize complex designs, make real-time adjustments, and refine models based on immediate feedback. This iterative process allows for faster development cycles, enabling manufacturers to bring products to market more quickly. Additionally, the ability to explore a wider range of design possibilities encourages innovation, allowing manufacturers to create cutting-edge products that were once thought to be unachievable. By enhancing accuracy, reducing costs, and fostering creativity, AI-driven modeling is transforming the landscape of manufacturing design, making it more efficient and effective than ever before.
Streamlining Production Processes with Predictive Analytics
Streamlining production processes through predictive analytics represents a major leap in manufacturing efficiency. By harnessing data from various sources—such as real-time sensors, historical production records, and sophisticated machine learning algorithms—predictive analytics allows manufacturers to anticipate potential disruptions before they occur. This proactive approach means that maintenance can be performed before equipment failures happen, reducing unplanned downtime and ensuring smooth operations. As a result, overall production efficiency is significantly enhanced, with fewer interruptions and more consistent output.
In addition to optimizing maintenance, predictive analytics plays a crucial role in refining supply chain management. By delivering precise demand forecasts, it enables manufacturers to accurately align inventory levels with anticipated needs. This foresight helps in adjusting production schedules and managing stock more effectively, minimizing the risks of both overstocking and shortages. Consequently, manufacturers benefit from a more responsive and flexible production system that not only reduces costs but also boosts customer satisfaction. Embracing predictive analytics allows manufacturers to improve operational efficiency, cut waste, and maintain a competitive edge in a rapidly evolving industry.
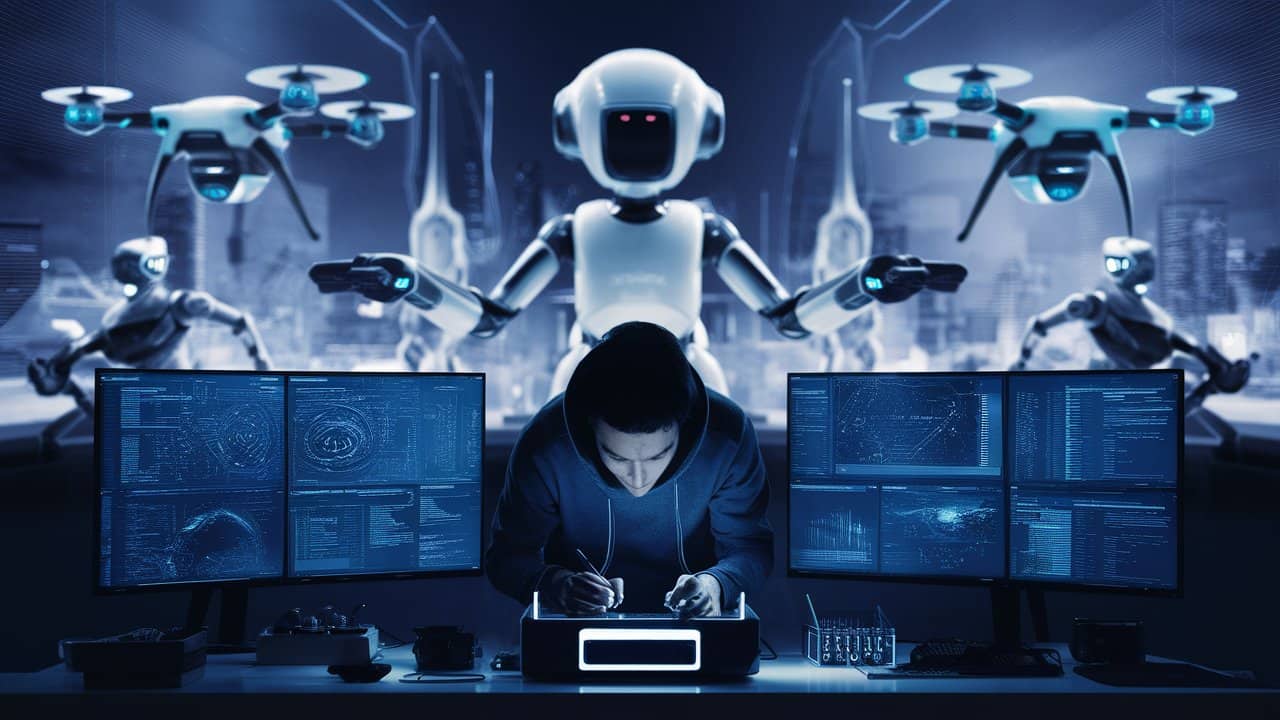
Quality Control Redefined: AI Inspection and Optimization
Quality control has always been a critical aspect of manufacturing, but traditional methods often struggle with inconsistencies and inefficiencies. The introduction of AI inspection and optimization represents a paradigm shift in how quality is maintained. AI-powered systems utilize advanced machine learning algorithms to inspect products with unprecedented accuracy. By analyzing images and sensor data, these systems can detect defects, deviations, and anomalies that might elude human inspectors. This not only enhances the precision of quality checks but also speeds up the inspection process, reducing the likelihood of costly recalls and ensuring higher standards of product excellence.
Moreover, AI-driven quality control systems can continuously learn and adapt over time. As they process more data, they refine their algorithms to improve detection capabilities and predict potential issues before they arise. This proactive approach enables manufacturers to address problems at their source, preventing defects from reaching the final stages of production. By integrating AI with existing quality control processes, companies can achieve a level of consistency and reliability that was previously unattainable.
The benefits of AI in quality control extend beyond mere detection. Optimization algorithms can analyze production data to identify trends and patterns that might indicate underlying issues. This data-driven insight allows manufacturers to fine-tune their processes, enhance operational efficiency, and minimize waste. As AI continues to evolve, its role in redefining quality control promises to drive innovation and set new benchmarks for excellence in manufacturing.
Benefits of AI in Manufacturing
Cost Reduction
The integration of AI into manufacturing processes heralds a new era of efficiency and cost-effectiveness. One of the most compelling advantages is cost reduction. Leveraging AI for predictive maintenance, optimizing resource allocation, and employing generative design techniques enables manufacturers to achieve substantial savings. Predictive maintenance algorithms, for instance, preemptively identify equipment issues, which minimizes downtime and costly repairs. Additionally, AI-driven resource optimization ensures that materials and energy are used efficiently, further cutting operational expenses.
Improved Product Quality
Another pivotal benefit is improved product quality. AI-powered quality control systems set a new standard for precision and consistency in manufacturing. These advanced systems detect even the slightest deviations or defects, ensuring that each product meets rigorous quality standards. By reducing human error and variability, AI enhances overall product reliability and customer satisfaction. Manufacturers can confidently deliver products that not only meet but exceed customer expectations, thereby bolstering their reputation in competitive markets.
Increased Productivity
Furthermore, AI enhances productivity through smart automation and streamlined processes. Automated systems powered by AI algorithms handle complex tasks with speed and accuracy, resulting in increased production output without compromising quality. By optimizing workflows and eliminating bottlenecks, manufacturers achieve higher throughput rates and quicker turnaround times. This not only boosts operational efficiency but also allows businesses to meet growing demand effectively. In essence, AI transforms manufacturing into a more agile and responsive industry, capable of adapting swiftly to market dynamics while maintaining high standards of productivity and quality.
Best Practices and Trends in Machine Learning for Product Engineering
Understanding the Intersection of Machine Learning and Product Engineering
Machine learning (ML) and product engineering are converging in transformative ways, revolutionizing traditional methodologies. At the intersection of these fields, AI-driven machine learning is automating complex tasks, optimizing processes, and enhancing decision-making. Product engineering, once heavily reliant on manual analysis and design, now leverages ML algorithms to predict outcomes, identify patterns, and improve efficiency. This synergy enables engineers to create more innovative, reliable, and cost-effective products.
For example, in the automotive industry, ML is utilized to enhance the engineering of self-driving cars. Traditional product engineering methods struggled with the vast array of data from sensors and cameras. By integrating machine learning, engineers can now process this data in real-time, allowing the vehicle to make split-second decisions. This not only improves the safety and functionality of self-driving cars but also accelerates development cycles, ensuring that advancements reach the market faster.
Current Trends in AI Applications for Product Development
1. Ethical AI:
Ethical AI focuses on ensuring that artificial intelligence systems operate within moral and legal boundaries. As AI becomes more integrated into product development, it’s crucial to address issues related to bias, fairness, and transparency. Ethical AI aims to create systems that respect user privacy, provide equal treatment, and are accountable for their decisions. Implementing ethical guidelines helps in building trust with users and mitigating risks associated with unintended consequences of AI technologies.
2. Conversational AI:
Conversational AI utilizes natural language processing (NLP) and machine learning to enable machines to comprehend and interact with human language naturally. This technology underpins chatbots and virtual assistants, facilitating real-time, context-aware responses. In product development, conversational AI enhances customer support, optimizes user interactions, and delivers personalized recommendations, resulting in more engaging and intuitive user experiences.
3. Evolving AI Regulation:
Evolving AI regulations are shaping product development by establishing standards for the responsible use of artificial intelligence. As AI technology advances, regulatory frameworks are being updated to address emerging ethical concerns, such as data privacy, bias, and transparency. These regulations ensure that AI systems are developed and deployed with safety and accountability in mind. For product development, adhering to these evolving standards is crucial for navigating legal requirements, mitigating risks, and fostering ethical practices, ultimately helping companies build trustworthy and compliant AI-driven products.
4. Multimodality:
Multimodality involves combining various types of data inputs—such as text, voice, and visual information—to create more sophisticated and effective AI systems. By integrating these diverse data sources, multimodal AI can enhance user interactions, offering richer and more contextually aware experiences. For instance, a product might utilize both voice commands and visual recognition to provide more intuitive controls and feedback.
In product development, this approach leads to improved usability and functionality. The integration of multiple data forms allows for a more seamless and engaging user experience, as it caters to different interaction preferences. By leveraging multimodal AI, companies can develop products that are not only more responsive but also better aligned with the diverse needs and behaviors of their users.
5. Predictive AI Analytics:
Predictive AI analytics employs machine learning algorithms to examine historical data and predict future trends or behaviors. This approach enables the analysis of patterns and trends within past data to forecast what might happen in the future. In product development, predictive analytics is invaluable for anticipating user needs, refining product features, and making informed, data-driven decisions.
By harnessing these insights, companies can significantly enhance product performance and streamline development processes. Predictive analytics allows for proactive adjustments and improvements, leading to reduced costs and increased efficiency. Moreover, by addressing potential issues and seizing opportunities before they arise, companies can boost user satisfaction and deliver products that better meet customer expectations.
6. AI Chatbots:
In product development, chatbots play a crucial role by enhancing user interaction and streamlining support processes. By integrating chatbots into customer service systems, companies can offer instant, accurate responses to user queries, manage routine tasks, and provide 24/7 support. This automation not only speeds up response times but also improves service efficiency and personalization, allowing businesses to address user needs more effectively. Additionally, chatbots can gather valuable data on user preferences and issues, which can inform product improvements and development strategies.
Implementing Machine Learning for Enhanced Product Design
Implementing machine learning in product design involves using advanced algorithms and data insights to enhance and innovate design processes. By analyzing large datasets, machine learning can reveal patterns and trends that improve design choices, automate tasks, and generate new ideas based on user feedback and usage data.
To integrate machine learning effectively, it’s essential to choose the right models for your design goals, ensure data quality, and work with cross-functional teams. Continuously refining these models based on real-world performance and user feedback will help achieve iterative improvements and maintain a competitive edge.
Future Outlook: The Role of Machine Learning in Product Innovation
The role of machine learning in future product innovation is poised for transformative change. As AI technologies advance, they will introduce more intelligent features that can adapt and respond to user behavior. Future innovations could lead to products that not only anticipate user needs but also adjust their functionalities dynamically, providing a more personalized and efficient experience.
Looking ahead, breakthroughs in AI, such as more advanced generative models and refined predictive analytics, will redefine product development. These advancements will allow companies to design products with enhanced capabilities and greater responsiveness to user preferences. By embracing these cutting-edge technologies, businesses will be well-positioned to push the boundaries of innovation, setting new standards and unlocking novel opportunities in their product offerings.
Digital Twins and IoT: Powering Smart Innovations
Imagine a bustling city where technology weaves an invisible web, responding to every citizen’s need with effortless precision. Traffic lights adjust intuitively, easing congestion before it forms. Energy grids anticipate demand spikes, seamlessly balancing supply to prevent outages. Public transport flows smoothly, routes adjusting in real-time to optimize commuter journeys. How is this possible? Meet the digital twins—virtual replicas of our physical world, meticulously crafted to mirror every detail.
Yet, these twins are not mere mirrors. They’re evolving with the help of Generative AI, transforming from static copies into dynamic problem-solvers. In high-tech factories, they predict machinery issues before they disrupt production, suggesting improvements that boost efficiency day by day. Across sprawling logistics networks, they forecast traffic and weather, guiding shipments to their destinations swiftly and on schedule.
This isn’t just progress; it’s a revolution. As Generative AI and digital twins integrate deeper into our lives, from city planning to healthcare, they’re reshaping industries with unprecedented innovation and operational prowess.
Integrating Generative AI with Digital Twins
Understanding the dynamics of IoT involves recognizing how integrating Generative AI with Digital Twins marks a profound transformation. Traditionally adept at real-time monitoring and simulation of physical assets or processes, Digital Twins now evolve into proactive decision-makers with the infusion of Generative AI.
Generative AI enhances Digital Twins by predicting behaviors and optimizing operations through comprehensive analysis of IoT-generated data. Imagine a manufacturing facility where Generative AI-powered Digital Twins not only replicate production lines but also predict maintenance needs and suggest process improvements autonomously. This collaboration significantly boosts operational efficiency by preemptively addressing challenges and optimizing resource usage.
This evolution represents more than just technical advancement; it marks a paradigm shift in how industries harness IoT capabilities. By leveraging Generative AI-enhanced Digital Twins, businesses can achieve unprecedented levels of efficiency and innovation. This advancement promises smarter, more adaptable systems within the IoT landscape, paving the way for transformative breakthroughs across diverse sectors.
Digital Twins in Action: Optimizing IoT Operations
Digital Twins are essential in IoT for enhancing operational efficiency across industries by replicating physical assets and systems, enabling real-time data simulation and insights. For example, in smart cities, Digital Twins adjust traffic flow using live IoT sensor data, optimizing urban mobility. In healthcare, they use predictive analytics to simulate patient scenarios, improving treatment and equipment maintenance. This proactive use minimizes downtime and maximizes resource efficiency, reducing costs and enhancing operations.
As IoT evolves and Digital Twins become more advanced, industries stand to benefit from significant innovations in efficiency and productivity. These integrated technologies promise transformative impacts, driving operational excellence across diverse sectors and paving the way for future advancements in IoT-driven solutions.
Smart Cities: Harmony Through Digital Twins
In the context of smart cities, digital twins revolutionize urban management by acting as virtual replicas of the city’s physical infrastructure. These digital counterparts meticulously simulate and monitor various aspects such as traffic patterns, energy usage, and public services like transportation. Powered by real-time data streamed from IoT sensors embedded throughout the city, digital twins facilitate agile decision-making and operational optimizations. For example, they can dynamically adjust traffic signal timings to alleviate congestion or reroute energy distribution to minimize waste. This proactive approach not only enhances urban efficiency and resource utilization but also improves the overall quality of life for residents. By integrating digital twins into urban planning and management, smart cities pave the way for sustainable growth and innovation, setting new standards for urban development in the digital age.
Predictive Insights: Leveraging Digital Twins in IoT Environments
In the world of IoT, Digital Twins emerge as powerful tools for predictive analytics, revolutionizing how industries optimize operations. These virtual counterparts of physical assets or processes continuously collect and analyze real-time data from IoT sensors. By harnessing this data, Digital Twins simulate various scenarios, predict future behaviors, and recommend proactive measures to enhance efficiency and performance.
Imagine a manufacturing plant where Digital Twins anticipate machinery failures before they occur, allowing for preemptive maintenance and minimizing production disruptions. In urban planning, Digital Twins can forecast traffic patterns based on historical and current data, facilitating better city management strategies. This predictive capability not only optimizes resource allocation but also fosters smarter decision-making across sectors, driving continuous improvement and innovation in IoT-enabled environments.
Applications of Digital Twins in IoT
Digital Twins are spearheading a transformative wave across industries within the IoT ecosystem. These virtual replicas of physical entities enable businesses to simulate real-world scenarios and optimize operations with unprecedented precision. In manufacturing, Digital Twins facilitate predictive maintenance, foreseeing equipment failures and optimizing production schedules to minimize downtime.
Moreover, in healthcare, Digital Twins simulate patient conditions to personalize treatment plans and predict health outcomes. Beyond these applications, Digital Twins are reshaping urban planning by modeling infrastructure performance and traffic flow, leading to more efficient city designs and management strategies. By leveraging Digital Twins, industries can achieve higher operational efficiency, reduced costs, and enhanced innovation, setting new benchmarks for performance and sustainability in the IoT era.
Implementing AI-driven Digital Twins in IoT
Implementing AI-driven Digital Twins in IoT environments presents both challenges and promising solutions. One major hurdle is the complexity of integrating diverse data streams from IoT devices into cohesive digital replicas. Ensuring seamless synchronization and real-time data processing is crucial for accurate predictive modeling and decision-making.
Moreover, maintaining data security and privacy while managing large volumes of sensitive information remains a critical concern. Solutions to these challenges include leveraging advanced AI algorithms for data fusion and anomaly detection, enhancing system interoperability through standardized protocols, and implementing robust cybersecurity measures to safeguard data integrity.
Successfully navigating these challenges enables businesses to harness the full potential of AI-driven Digital Twins. They empower organizations to achieve operational efficiencies, predictive insights, and innovation across sectors, shaping the future of IoT applications with intelligent and responsive systems.
IoT is undeniably the backbone of digital twins, forming the essential foundation upon which these advanced systems are built. Through the vast network of interconnected devices, IoT provides the real-time data necessary for creating accurate and dynamic digital replicas. This continuous data flow is crucial for the functionality of digital twins, as it allows for constant monitoring and updating of physical assets.
Advanced data analytics and AI utilize this data to generate actionable insights and predictive maintenance strategies. Cloud computing ensures seamless storage and processing of vast amounts of data, enabling real-time decision-making. By recognizing IoT as the core component, we acknowledge its pivotal role in harmonizing various technologies. This integration empowers digital twins to drive efficiency, innovation, and transformation across multiple industries. Thus, IoT stands as the cornerstone, unlocking the full potential of digital twin technology.
Top 5 Strategies AWS Partners Use to Leverage AWS Infrastructure for Generative AI
Discover the transformative power of AWS in scaling generative AI. From groundbreaking networking advancements to revolutionary data center strategies, AWS is continuously enhancing its infrastructure. These innovations not only bolster the capability but also redefine the scalability of generative AI solutions. Embrace a future where AWS sets the benchmark in cloud-based technologies, empowering businesses to harness the full potential of artificial intelligence at unprecedented scales.
Generative artificial intelligence (AI) has rapidly revolutionized our world, enabling both individuals and enterprises to enhance decision-making, transform customer experiences, and foster creativity and innovation. However, the robust infrastructure supporting this powerful technology is the culmination of years of innovation. This sophisticated foundation allows generative AI to thrive, demonstrating that behind every breakthrough is a history of dedicated advancement and development. In this blog, we’ll explore the top five strategies AWS partners use to maximize AWS infrastructure for generative AI, explained in a way that anyone can understand.
1. Harnessing Low-Latency, High-Performance Networking
Generative AI models rely on massive amounts of data to learn and generate accurate predictions. Efficiently managing and processing this data requires advanced networking technologies that facilitate fast and reliable data movement across the cloud infrastructure. AWS partners leverage these specialized networking solutions to optimize performance and enhance the capabilities of their generative AI applications.
Elastic Fabric Adapter (EFA): EFA acts as a super-fast highway for data, enabling rapid data transfer by bypassing traditional network bottlenecks. When training generative AI models, which often involves processing large datasets and requiring frequent communication between multiple servers, EFA ensures data reaches its destination swiftly. This accelerated data movement is crucial for training complex AI models efficiently.
Scalable Reliable Datagram (SRD): SRD functions like a high-speed courier service for data packets, ensuring quick and reliable delivery. Working in tandem with EFA, SRD guarantees that data packets are not only transferred rapidly but also consistently, which is vital for maintaining the accuracy and performance of AI models. This combination of speed and reliability is essential for efficient model training and inference.
UltraCluster Networks: Imagine a vast network of interconnected supercomputers, each linked by ultra-fast and dependable cables. UltraCluster Networks are designed to support thousands of high-performance GPUs (graphics processing units), providing the computational power needed for training large-scale generative AI models. These networks offer ultra-low latency, meaning there is minimal delay in data transfer, significantly accelerating the training process and enabling faster model iterations.
2. Enhancing Energy Efficiency in Data Centers
Operating AI models demands substantial electrical power, which can be costly and environmentally impactful. AWS partners leverage AWS’s advanced data centers to boost energy efficiency and reduce their environmental footprint.
Innovative Cooling Solutions: Data centers house thousands of servers that generate considerable heat during operation. AWS employs advanced air and liquid cooling technologies to efficiently regulate server temperatures. Liquid cooling, resembling a car’s radiator system, effectively manages heat from high-power components, significantly lowering overall energy consumption.
Environmentally Responsible Construction: AWS prioritizes sustainability by constructing data centers with eco-friendly materials such as low-carbon concrete and steel. These materials not only diminish environmental impact during construction but also throughout the data centers’ operational life. This commitment helps AWS partners in cutting down carbon emissions and promoting environmental responsibility.
Simulation and Optimization: Prior to constructing a new data center, AWS conducts detailed computer simulations to predict and optimize its performance. This simulation-driven approach enables AWS to strategically place servers and cooling systems, maximizing operational efficiency. Similar to planning a building’s layout in a virtual environment, this ensures minimal energy usage and operational costs while maintaining optimal performance.
3. Ensuring Robust Security
Security is paramount for AWS partners, particularly when handling sensitive data essential for generative AI models. AWS implements a suite of advanced security measures to protect data and ensure compliance with stringent regulations.
AWS Nitro System: Serving as a vigilant guardian, the AWS Nitro System enforces rigorous isolation between customer workloads and AWS infrastructure. It features secure boot capabilities that prevent unauthorized software from executing on servers, thereby maintaining data integrity and confidentiality.
Nitro Enclaves: Within servers, Nitro Enclaves establish secure, isolated environments. Integrated with AWS Key Management Service (KMS), they encrypt data during processing to create a secure enclave for sensitive information, analogous to a digital safe, shielding it from exposure.
End-to-End Encryption: AWS employs robust encryption methods to secure data both at rest and in transit across its infrastructure. This comprehensive approach ensures data remains protected with stringent access controls, bolstering security against unauthorized access.
Compliance and Certifications: AWS adheres strictly to global security standards and holds numerous certifications, underscoring its commitment to data protection and regulatory compliance. These certifications reassure customers of AWS’s capability to safeguard their data with the highest security measures in place.
4. Harnessing Specialized AI Chips
Efficient operation of AI models relies heavily on specialized hardware. AWS partners harness purpose-built AI chips from AWS to optimize the performance and cost-effectiveness of their generative AI applications.
Strategic Collaborations: AWS collaborates closely with industry leaders such as NVIDIA and Intel to provide a diverse range of accelerators. These collaborations ensure that AWS partners have access to cutting-edge hardware tailored to their specific AI needs.
Continuous Innovation: AWS continues to lead in AI hardware development. For example, the upcoming Trainium2 chip promises even faster training speeds and improved energy efficiency. This ongoing innovation enables AWS partners to maintain a competitive advantage in the dynamic field of AI.
5. Enhancing Scalability in AI Infrastructure
Scalability is crucial for the success of generative AI applications, which often face unpredictable computing demands. AWS provides a versatile and resilient infrastructure that empowers partners to dynamically adjust resources to meet evolving requirements.
Auto Scaling: AWS’s Auto Scaling feature automatically adjusts computing resources based on application demand. When an AI workload requires more processing power, Auto Scaling efficiently adds servers to maintain optimal performance. This capability ensures consistent application responsiveness and efficiency, supporting uninterrupted operations.
Elastic Load Balancing (ELB): ELB evenly distributes incoming traffic across multiple servers to prevent any single server from becoming overwhelmed. By intelligently distributing workloads, ELB optimizes resource allocation, enhancing the overall performance and reliability of AI applications. This ensures seamless operation even during periods of peak usage.
Amazon S3 (Simple Storage Service): S3 offers scalable storage solutions for securely storing and retrieving large volumes of data as needed. Acting as a flexible digital repository, S3 effectively manages diverse data requirements, seamlessly supporting the storage and retrieval needs of AI applications.
Amazon EC2 (Elastic Compute Cloud): EC2 provides resizable compute capacity in the cloud, enabling partners to deploy and scale virtual servers rapidly in response to fluctuating workload demands. This flexibility is crucial for iterative model testing, experimentation, and efficient scaling of production environments, facilitating agile development and deployment of AI applications.
Conclusion
AWS Partner Companies are leveraging AWS’s advanced infrastructure to push the boundaries of what’s possible with generative AI. By utilizing low-latency networking, enhancing energy efficiency, ensuring robust security, leveraging specialized AI chips, and implementing scalable infrastructure, they can deliver high-performance, cost-effective, and secure AI solutions. These strategies not only help in achieving technological advancements but also ensure that AI applications are sustainable and accessible to a wide range of industries. As generative AI continues to evolve, AWS and its partners will remain at the forefront, driving innovation and transforming how we interact with technology.
AWS Solutions for Healthcare Data Migration Challenges
No industry is without its challenges, and the healthcare sector is no exception, particularly when it comes to using cloud services for data migration. In depth of discussing the
What is healthcare data migration?
Healthcare data migration involves transferring extensive volumes of medical records, patient information, and various health-related data from one system to another. This complex process is vital for healthcare organizations transitioning to more advanced information systems, consolidating data from diverse sources, or adopting cloud-based solutions. As healthcare systems evolve, the ability to manage and transfer data efficiently becomes crucial. Migration ensures that all historical and current patient data are preserved and remain accessible in new systems, which is critical for delivering continuous and quality care. It also addresses the need to consolidate disparate data sources into a unified platform, simplifying data management, and improving the overall efficiency of healthcare operations.
Why is it needed?
The need for data migration in healthcare stems from several key objectives. First and foremost, it aims to enhance patient care by ensuring healthcare providers have immediate and comprehensive access to patient histories, enabling informed decision-making. Additionally, it facilitates the adoption of advanced data analytics tools, which can uncover insights for better clinical outcomes and operational efficiencies.
Data migration is also crucial for meeting regulatory requirements, such as data privacy laws and standards, ensuring compliance and protecting patient information. Effective data migration supports interoperability, allowing different healthcare systems and applications to work together seamlessly. This reduces the risk of data loss during transitions and helps healthcare organizations keep pace with technological advancements and evolving industry standards, ultimately leading to more resilient and adaptable healthcare infrastructures.
Navigating Data Migration Phases: An In-Depth Guide
Planning and Assessment
The initial phase of planning and assessment is crucial for comprehensively identifying all pertinent data sources, thereby grasping the migration scope. Clear migration objectives are set to establish precise goals, while estimating downtimes aids in mitigating operational disruptions. Moreover, selecting appropriate migration mediums and delineating project timelines ensures a structured and orderly approach to the migration process, thereby facilitating a seamless transition.
Data Profiling and Cleansing
In the data profiling and cleansing phase, a meticulous examination of data is undertaken to evaluate its format and quality thoroughly. It involves identifying and rectifying duplicate, corrupted, or inconsistent data to uphold data integrity. This step is fundamental to ensuring the accuracy and dependability of migrated data in the new system, effectively mitigating the risk of transferring flawed information.
Data Transformation and Mapping
Data transformation and mapping involve adapting data formats to align with the requirements of the target system. Guaranteeing compatibility and consistency between source and target databases is essential for seamless data integration. Additionally, remapping the data structure to correspond with the target database schema contributes to maintaining data integrity throughout the migration process.
Data Migration Execution:
Following the completion of planning and preformatting, the actual data migration and synchronization commence. This phase encompasses transferring data from the source to the target system while minimizing disruptions to ongoing operations. Continuous monitoring and validation play a pivotal role in promptly identifying and resolving any issues or errors, thereby ensuring a successful transition to the new system with precise and readily accessible data.
Challenges facing while migrating the data and their solutions
Meeting Compliance Standards
The introduction of new technologies in the healthcare sector presents a significant hurdle in adhering to data privacy regulations such as HIPAA. Compliance with these regulations is especially challenging during migrations due to the transfer of protected health information (PHI).
Ensuring compliance entails securely transferring data via encryption and vendor compliance assurance. This involves encrypting the data entirely and verifying that the database vendor meets HIPAA compliance standards. Furthermore, it’s crucial to monitor user access to the data by implementing logging measures that capture all user interactions.
Risk of Data Breaches
Data breaches pose a critical concern during healthcare data migration on AWS due to the sensitive nature of healthcare data. Cyber attackers are attracted to this valuable information, aiming for financial gain or malicious intent. To safeguard patient information throughout the migration process, implementing security protocols like encryption and continuous monitoring is essential, reducing the risk of compromising patient privacy.
Furthermore, comprehensive data breach response plans are vital for healthcare data migration on AWS. Despite preventive measures, breaches may occur, requiring effective response mechanisms. Well-defined response plans enable organizations to promptly mitigate the impact of breaches, minimizing potential damage to operations. Proactive management of data breach risks enhances security and ensures the protection of patient data during migration on AWS.
Data Interoperability
Achieving seamless data interoperability between various healthcare systems and applications poses a significant challenge during AWS data migration. The presence of disparate data formats, structures, and standards complicates the integration and exchange of data, necessitating meticulous data mapping and standardization efforts. These complexities can impede the smooth transition of information between systems, hindering efficient healthcare operations and patient care delivery.
To address these challenges, the adoption of interoperability standards such as HL7 plays a pivotal role in facilitating seamless communication and interoperability among systems. By adhering to established standards, healthcare organizations can streamline data exchange processes and enhance the compatibility of disparate systems. Leveraging HL7 and similar standards ensures that data can be accurately interpreted and exchanged, promoting efficient collaboration and information sharing across the healthcare ecosystem.
Cost Optimization
Cost optimization is a major challenge in healthcare data migration on AWS due to the significant expenses linked to transferring large data volumes. Achieving cost efficiency demands meticulous planning and strategy optimization. Healthcare organizations can ease the financial burden by adopting best practices and utilizing AWS cost management tools. These tools enable efficient resource monitoring, identification of cost-saving opportunities, and implementation of budget-friendly solutions.
Employing strategies like data compression and AWS Storage Gateway can minimize expenses during migration. Furthermore, optimizing the selection of AWS storage services based on data usage patterns can further cut costs. Leveraging AWS’s scalable infrastructure allows healthcare organizations to streamline migration processes and meet their cost optimization goals effectively.
Planned Downtime
Minimizing planned downtime in healthcare data migration is vital for uninterrupted access to patient information. Careful planning and scheduling of migration activities are essential to avoid disruptions to clinical workflows. Healthcare organizations can strategically schedule tasks during low-traffic periods to minimize operational impact. Implementing failover mechanisms and redundancy measures ensures service availability during migration. These strategies collectively enable healthcare providers to access patient data seamlessly, safeguarding continuity of care.
Data replication ensures real-time data syncing between systems, facilitating seamless failover during migration. Phased migration breaks down the process into manageable stages, maintaining service continuity. Combining these strategies with meticulous planning minimizes planned downtime in healthcare data migration. Thus, uninterrupted access to patient information and healthcare services is upheld efficiently.
Data Analysis
Effective data analysis is vital for extracting valuable insights from healthcare data migrated to AWS. Robust analytics capabilities empower healthcare organizations to improve patient outcomes and optimize healthcare delivery. Leveraging AWS analytics services like Amazon Redshift and Amazon QuickSight enables efficient analysis of large data volumes, facilitating informed decision-making to enhance patient care.
By utilizing these advanced tools, healthcare organizations can uncover trends, patterns, and correlations within their data, facilitating targeted interventions and personalized treatment plans. AWS analytics services provide scalability and flexibility, allowing organizations to manage growing data volumes and adapt to changing healthcare demands seamlessly.
In a nutshell, migrating healthcare data onto AWS comes with its fair share of hurdles, spanning regulatory compliance, security concerns, and cost management. Nevertheless, through thorough planning, stringent security protocols, adherence to industry standards, and tapping into AWS’s analytics tools, healthcare institutions can navigate these obstacles successfully. By carefully strategizing, following established guidelines, and making the most of AWS offerings, the transition of healthcare data can lead to improved patient services, streamlined operations, and adaptability in the dynamic healthcare sector.