Smart Search: AI Engines Redefining Digital Discovery
AI-powered search engines have intensified information retrieval, marking a significant advancement in user experience. Traditional search engines relied on basic algorithms and keyword matching, struggling to keep up with the growing complexity and volume of digital content. The introduction of AI-driven platforms ushered in a new era of search by utilizing machine learning and natural language processing (NLP) to grasp user intent, resulting in personalized search results and greater contextual relevance. Perplexity AI, Bing, YEP, and You.com are among the platforms leveraging these technologies to enhance search capabilities, with Perplexity AI excelling in interpreting complex queries and Bing offering personalized, conversational experiences.
This transformation in search technology not only reshapes how users access information but also revolutionizes their engagement with digital platforms. AI-powered engines now deliver more intuitive and personalized outcomes, tailored to individual behaviors and preferences. As AI progresses, these platforms are set to play a more significant role in providing precise, context-aware, and ethical digital experiences. This evolution suggests a future where search engines comprehend user intent better, paving the way for a personalized and responsible digital environment.
The Evolution from Traditional to AI-Powered Search
The shift from traditional to AI-powered search engines has revolutionized how we access digital information. Early search engines relied on basic algorithms and keyword matching, which often produced inaccurate results due to their inability to grasp deeper context or user intent. In contrast, AI-powered search engines utilize machine learning and natural language processing (NLP) to interpret context, semantics, and user behavior, providing more accurate and personalized search experiences.
AI’s integration has significantly enhanced search engine capabilities, enabling a better understanding of user intent and more tailored results. This evolution sets a new standard for accuracy and relevance while ushering in the future of predictive, conversational, and voice-activated searches, ultimately transforming the user experience and redefining the digital search landscape.
NLP Enhances Searches; Machine Learning Boosts Precision
The utilization of Natural Language Processing (NLP) is instrumental in enhancing search functionality as it enables search engines to effectively comprehend and interpret user queries. Through the processing of intricate language structures, NLP facilitates the delivery of more precise and contextually pertinent search results, ultimately enriching the user experience by fostering intuitive and conversational interactions that bridge the gap between human language and machine understanding.
Simultaneously, machine learning algorithms play a pivotal role in further enhancing search precision by continuously assimilating insights from user interactions and behaviors. By scrutinizing extensive datasets to identify patterns and trends, these algorithms empower search engines to optimize results in alignment with individual preferences and intent. The combined prowess of NLP and machine learning engenders a formidable synergy that elevates search capabilities, yielding highly personalized outcomes and reshaping the dynamics of user access and engagement with online information. This evolution paves the way for a new standard of accuracy and relevance within the digital search landscape.
Understanding User Intent: How AI Personalizes Search Results
In the domain of AI-driven search engines, the ability to discern user intent is crucial for delivering personalized search results. Through meticulous analysis of keywords, contextual hints, and user behavior, AI algorithms can accurately grasp the true purpose behind each search query. By employing advanced techniques in natural language processing (NLP) and machine learning, these algorithms can predict user preferences and tailor search results accordingly, ensuring relevance and elevating user satisfaction.
By continuously learning from user interactions, AI not only hones its understanding of intent but also anticipates future needs, offering a more intuitive and tailored browsing experience. This proactive approach not only simplifies information retrieval but also promotes deeper user engagement by presenting content that closely matches individual preferences and browsing habits. As AI progresses, its ability to interpret and cater to user intent is poised to revolutionize how people interact with and consume digital information.
The Role of AI in Voice and Visual Search
Voice and visual search are advanced search technologies that allow users to find information through spoken commands or images, rather than traditional text-based queries. Voice search uses speech recognition to interpret and respond to voice queries, making it easier for users to interact with search engines hands-free. Visual search enables users to upload or capture images, allowing AI to analyze and identify objects, text, or scenes within the image for relevant search results.
AI plays a vital role in both technologies. In voice search, AI uses NLP to understand context and intent, delivering accurate and relevant responses. For visual search, AI leverages machine learning and computer vision to recognize patterns, objects, and features in images. This helps users explore information more intuitively, enhancing personalization and improving overall search accuracy and efficiency.
The Future of Search: What’s Next for AI-Powered Engines?
The future looks promising for AI-powered search engines, with continuous developments in natural language processing, machine learning, and predictive analytics driving them towards predictive and personalized search interactions. By enhancing their ability to understand user intent, context, and preferences, AI-driven search engines are poised to deliver highly tailored results, heralding a new era focused on precision and relevance in search experiences.
Anticipated improvements in voice-activated searches and conversational interfaces are expected to revolutionize user experiences, facilitating more fluid and intuitive interactions with search platforms. This shift towards user-friendly interfaces foresees a future where individuals can engage with search engines in a natural, conversational manner, fundamentally altering the landscape of information retrieval. Furthermore, a spotlight on AI ethics and transparency will be essential to address bias and ensure responsible AI practices, paving the way for search engines that offer personalized, accurate, and ethically sound digital experiences.
Legacy Chatbot vs AI Chatbot: Which is the Better Choice?
Legacy chatbots are built on older technology, using rule-based systems that rely on predefined scripts and keywords to handle interactions. They work well for basic queries but struggle with more complex conversations, often limiting customer engagement and satisfaction. Legacy chatbots include scripted responses, heavy reliance on keyword recognition, and basic functionality for tasks like answering FAQs. They also lack learning capabilities, meaning they don’t improve with each interaction, which can lead to repetitive and limited responses.
AI chatbots have revolutionized the chatbot landscape by using natural language processing (NLP), machine learning, and advanced algorithms. They offer more personalized and meaningful interactions, adapting to user needs and providing efficient responses. AI chatbots include understanding natural language for human-like conversations, learning from past interactions to improve, maintaining context during exchanges, and handling diverse tasks such as customer support and sales inquiries.
AI Innovations: Enhancing Customer Service & Productivity
Enhancing Customer Experience with AI-Powered Virtual Agents and Voice Assistants
Customer service virtual agents and voice assistants leverage generative AI to revolutionize customer interactions. These innovative technologies offer intuitive conversational interfaces that enhance human capabilities and cater to diverse business needs.
Boosting Enterprise Productivity through AI-Powered Solutions
By incorporating generative AI-powered chatbots, enterprises can standardize internal communications and empower employees with self-service options for common inquiries. Moreover, these solutions improve conversation flows for tech support and streamline routine activities, ultimately reducing human error rates.
Benefits of AI Chatbots
- Conversational AI: AI chatbots use advanced NLP to engage in human-like interactions, making customers feel like they are talking to a real person. This personalized experience fosters meaningful interactions, addressing customer concerns with accuracy and empathy.
2. Empowering Self-Service: AI chatbots provide intelligent self-service options that efficiently handle a wide range of inquiries, streamlining the support process and saving time for customers. This enhances convenience and overall satisfaction, reducing the burden on human agents and improving the customer journey.
3. Cost-Effective Support: AI chatbots automate repetitive tasks, like answering common questions, reducing the need for human agents and resulting in substantial cost savings for businesses. Additionally, they provide 24/7 customer support, ensuring service availability without incurring the costs of constant staffing.
User Experience with AI Chatbots
AI chatbots excel in providing a smooth and pleasant user experience by adeptly comprehending natural language, retaining context, and customizing interactions. Through the analysis of user data, they can customize responses, suggestions, and deals according to individual preferences, elevating the degree of personalization. Moreover, AI chatbots offer constant support, guaranteeing that customers can receive help at any time, in contrast to human agents. Furthermore, they enhance efficiency by managing numerous inquiries concurrently, cutting down on wait times and notably increasing response rates, ultimately streamlining and enhancing the overall customer experience.
Choosing the Right Chatbot for Business
When it comes to selecting a chatbot for business, the choice between legacy and AI chatbots hinges on the specific needs, objectives, and budget. Each option has its strengths and weaknesses, so it’s important to weigh various factors before making a decision. Below are some key considerations that can guide choosing the right chatbot solution:
1. Business Objectives
Understanding business goals is critical for selecting the most suitable type of chatbot. For companies with short-term objectives, such as quickly managing simple customer inquiries, answering frequently asked questions, or guiding users through basic processes, a legacy chatbot may suffice. These chatbots can efficiently handle straightforward tasks without the need for complex technology. However, for businesses focused on long-term growth and improving customer engagement, investing in an AI chatbot is a more advantageous option.
AI chatbots provide enhanced features, including personalized responses and contextual understanding, which facilitate deeper customer relationships and lead to greater satisfaction. This makes AI chatbots valuable for businesses aiming to scale their operations and enhance overall customer experience.
2. Customer Expectations
Customer expectations play a crucial role in choosing the right chatbot. If the customer base prefers quick, straightforward responses with minimal interaction, a legacy chatbot may be ideal for handling basic queries efficiently. However, if a more personalized and engaging experience is expected, AI chatbots are the better option, as they can understand natural language, adapt to individual preferences, and provide dynamic interactions that enhance customer satisfaction.
3. Budget Constraints
Legacy chatbots tend to have lower initial costs, which can be attractive for businesses with tight budgets, but they may require more maintenance over time, increasing overall expenses. AI chatbots, while requiring a higher upfront investment, offer significant long-term savings by managing a greater volume of inquiries, boosting efficiency, and improving customer satisfaction, ultimately delivering a stronger return on investment.
4. Technical Infrastructure
Assessing the current technical setup is vital for maintaining smooth operations. It’s important to determine how effectively a chatbot can integrate with existing systems like customer relationship management (CRM) software and other business tools. AI chatbots are typically built for compatibility with modern platforms, enabling seamless integration with various applications, which improves overall operational performance.
5. Scalability Requirements
Anticipating growth or an increase in customer inquiries makes opting for an AI chatbot a wise decision. These chatbots are designed to scale, allowing them to adapt to changing demands and handle more complex queries as the business expands. This scalability ensures that customer service capabilities grow in tandem with the business, facilitating the maintenance of high-quality service levels.
In a nutshell, AI chatbots have many benefits when comparing legacy chatbots and AI chatbots. They are more capable, provide a better user experience, and can easily adapt to different situations. While legacy chatbots can be useful for specific tasks, their limitations can lead to unhappy customers, especially in today’s fast-paced digital world.
As companies focus more on keeping customers engaged and running their operations smoothly, AI chatbots are becoming the preferred option. By recognizing the differences between the two chatbot types and aligning the choice with business objectives, organizations can make the best selection for their needs. Ultimately, investing in an AI chatbot can enhance customer interactions, support business growth, and help succeed in the digital age.
Overcoming Telecom Management Challenges with Generative AI
The Telecommunications Management Network (TMN) is an architectural framework designed to manage network resources effectively through predefined interfaces, now enhanced by AI technologies. This hierarchical approach allows for efficient modeling of network resources and management entities, addressing various aspects of telecommunications, from operations to service and business management, while leveraging AI to optimize performance and streamline communication.
TMN’s architecture consists of several key layers. The element management layer utilizes AI for monitoring lower-level resources like switching systems, enhancing performance management, and metrics tracking. The network management layer employs AI to analyze data for improved connection management and resource grouping. In the service management layer, AI enhances service logic, ensuring efficient resource utilization. Finally, the business management layer integrates AI to optimize stakeholder relationships and oversee service deployment from a commercial perspective.
AI Integration for Enhanced Customer Service in Telecom
In the rapidly evolving telecommunications sector, leveraging AI in customer service has become essential for staying competitive. As customer expectations rise, telecom companies must deliver prompt support to ensure loyalty. AI-driven solutions like chatbots, virtual assistants, and automated communication services enable round-the-clock inquiry management, significantly cutting wait times and providing immediate assistance, thereby elevating the overall customer experience.
AI’s capability to analyze customer interactions reveals valuable insights into behavior patterns. This understanding allows telecom providers to customize their services effectively, proactively addressing issues and minimizing churn while fostering stronger customer loyalty.
Ultimately, integrating AI not only enhances operational efficiency but also equips telecom companies to swiftly respond to market changes. By delivering personalized service tailored to individual preferences, telecom providers can deepen customer relationships and achieve long-term success in telecommunications management.
Minimizing Downtime: Predictive Maintenance with AI
Minimizing downtime in telecom operations is critical, and predictive maintenance powered by AI offers a robust solution. By analyzing real-time data from network equipment and infrastructure, AI can predict potential failures before they occur. This proactive approach enables telecom companies to schedule maintenance precisely when needed, rather than relying on reactive measures that can lead to unexpected outages.
Additionally, predictive maintenance enhances asset management by optimizing resource allocation. AI algorithms can identify patterns and trends that human operators might overlook, allowing for timely interventions that extend the life of critical infrastructure. By reducing the frequency and duration of equipment failures, telecom providers not only maintain seamless service for their customers but also significantly cut maintenance costs.
Ultimately, integrating AI for predictive maintenance empowers telecom companies to transform their operational strategies. This shift not only minimizes downtime but also boosts overall efficiency, leading to improved customer satisfaction and loyalty. By embracing this technology, firms can ensure their networks remain resilient against the demands of an increasingly digital world.
AI-Enhanced Resource Management in TMN
AI-enhanced resource management in the Telecommunications Management Network (TMN) is vital for optimizing complex telecom infrastructures. By leveraging AI technologies, operators can analyze real-time data, facilitating better decision-making and resource allocation. This proactive management minimizes downtime and enhances service reliability, allowing telecom companies to respond swiftly to potential issues and optimize network performance effectively.
In the broader scope, this integration significantly boosts customer satisfaction. Improved network performance and reduced service interruptions create a seamless experience for users, which is crucial in today’s competitive market. AI-driven insights enable telecom operators to adapt to fluctuating demands and ensure efficient service delivery, ultimately strengthening customer loyalty.
Moreover, AI-enhanced resource management fosters organizational agility, equipping telecom companies to embrace emerging technologies like 5G and IoT. This adaptability not only streamlines day-to-day operations but also positions operators for future growth. By integrating AI within TMN, telecom providers become more resilient and responsive, ready to tackle evolving industry challenges and capitalize on new opportunities.
AI for Streamlined Data Management and Traffic Forecasting
AI is crucial for streamlined data management and traffic forecasting in the telecommunications industry due to the exponential growth of data and the complexity of network operations. As networks expand and user demands increase, telecom operators face challenges in efficiently managing vast amounts of data while ensuring optimal performance. AI-driven analytics tools can process and analyze this data in real-time, allowing operators to make informed decisions quickly. By leveraging machine learning algorithms, AI can identify patterns in traffic usage and predict future demand, enabling proactive adjustments to network resources.
Moreover, accurate traffic forecasting is essential for maintaining service quality and customer satisfaction. AI can enhance forecasting models by incorporating various factors such as historical usage data, time-of-day trends, and external influences like events or weather conditions. This predictive capability allows telecom operators to allocate resources effectively, minimizing congestion and ensuring smooth service delivery.
Ultimately, the integration of AI in data management and traffic forecasting not only improves operational efficiency but also enhances the overall user experience. By anticipating user needs and optimizing network performance, telecom providers can maintain a competitive edge in a rapidly evolving market, ensuring reliable connectivity for their customers.
The Transformative Impact of Generative AI in Telecom
GenAI is revolutionizing the telecom industry as networks grow more complex with the implementation of 5G and IoT. Efficiently managing these networks is increasingly challenging, but GenAI addresses this by automating tasks, optimizing performance, and enhancing the customer experience. By predicting network traffic patterns, telecom providers can proactively allocate resources, effectively reducing congestion and downtime while improving overall operational efficiency.
In addition to network management, GenAI is significantly enhancing customer support. AI-powered chatbots and virtual assistants enable telecom companies to handle inquiries more efficiently, offering real-time assistance that eases the burden on human agents. This not only boosts customer satisfaction but also streamlines support processes. As GenAI continues to drive innovation, it will facilitate personalized services, bolster security measures, and enable seamless network management, positioning telecom companies at the forefront of technological advancements. The influence of GenAI in the telecom sector is profound and essential for future competitiveness.
The Impact of Generative AI on Real Estate Document Processing
How AI is Automating Real Estate Paperwork?
AI is transforming the real estate industry by automating paperwork handling through intelligent document processing (IDP). This technology utilizes AI, machine learning, and natural language processing to streamline the management of documents such as contracts, lease agreements, and property records. By automating these tasks, IDP enhances efficiency and accuracy, significantly reducing the manual effort required for document management.
The IDP process begins with document ingestion, where files are captured from various sources like emails, scanners, or cloud storage and converted into digital formats if necessary. Preprocessing follows, which includes noise reduction, image correction, and text extraction to prepare the documents for accurate data extraction. During the data extraction phase, AI algorithms analyze the content to identify and pull out specific information such as names, dates, and contract terms, using natural language processing to understand the text’s context.
Once the data is extracted, it undergoes validation to ensure accuracy by cross-referencing with existing databases or predefined rules. The validated data is then classified into relevant categories, such as lease agreements or property sale contracts. Finally, the classified data is integrated into systems or databases, such as property management or CRM platforms. A review stage, involving human oversight, ensures data accuracy and provides feedback to refine AI models, resulting in a more efficient and reliable document processing system for real estate professionals.
AI’s Role in Streamlining Real Estate Transactions
AI is transforming the real estate industry by boosting efficiency and lowering costs. By automating crucial tasks like document management, contract review, and compliance checks, AI reduces the need for manual effort and minimizes errors. This acceleration in processing speeds up transactions, allowing both real estate professionals and clients to finalize deals more swiftly and accurately. With AI handling these routine tasks, agents can concentrate on building client relationships and strategic planning, ensuring a more seamless transaction experience.
The financial benefits of AI in real estate are significant. Automation cuts down on the need for extensive human resources, which can otherwise lead to higher operational costs. By streamlining workflows and speeding up decision-making, AI enables real estate firms to manage transactions more efficiently. This results in substantial savings on administrative expenses and allows businesses to use their resources more effectively, enhancing their bottom line.
Additionally, AI’s ability to process information rapidly leads to faster deal closures and improved cash flow. By reducing manual intervention and optimizing workflows, AI helps real estate firms close transactions more quickly, resulting in timely revenue generation. This efficiency decreases operational costs and provides a competitive edge, allowing businesses to stay ahead in a dynamic market. Adopting AI technology equips real estate firms to navigate industry changes with agility and precision.
Data Security in Automated Document Processing with AI
In this real estate sector, AI-driven automated document processing enhances both efficiency and data security. By integrating advanced encryption and secure access controls, AI systems protect sensitive information, such as contracts and client records, from unauthorized access and breaches. This robust security framework ensures the confidentiality of data and builds trust with clients.
AI also boosts security through continuous monitoring of anomalies and potential threats. This real-time threat detection provides an extra layer of protection, allowing real estate professionals to manage documents efficiently while maintaining high standards of data security. With AI, businesses can streamline operations without compromising on data integrity.
Reducing Errors: The Accuracy of AI-Driven Documentation
AI-driven documentation is revolutionizing the real estate sector by significantly reducing errors in paperwork. Traditional methods often involve manual data entry and review, which can lead to mistakes, delays, and costly disputes. However, AI systems, equipped with machine learning algorithms, can automatically extract, analyze, and verify large volumes of data with a high degree of accuracy. This not only eliminates the risk of human error but also ensures compliance with legal standards, which is critical in real estate transactions. As a result, real estate professionals can experience smoother and faster approval processes.
Moreover, AI’s ability to review documents in real-time allows for the instant identification of inconsistencies and omissions that could otherwise be overlooked. By flagging potential issues early, AI solutions minimize the need for time-consuming corrections and reduce the chance of legal complications down the line. This enhanced accuracy and efficiency lead to greater trust between stakeholders, allowing agents, buyers, and sellers to focus on the deal rather than on documentation errors, ultimately improving the overall real estate transaction experience.
AI-Powered Document Review: Faster Approvals and Compliance Checks
AI-powered document review is transforming the real estate sector by automating critical tasks such as contract verification and compliance checks. Using advanced machine learning algorithms, AI swiftly identifies errors, missing information, and inconsistencies within documents, ensuring they meet regulatory standards. This not only reduces the time required for approvals but also minimizes the chances of human error, making transactions more efficient. Real estate professionals benefit from the speed and accuracy of AI, allowing them to close deals faster. As a result, the process becomes more streamlined, improving productivity across the board.
In addition to speeding up approvals, AI significantly reduces legal risks by ensuring compliance with the latest regulations. It continuously monitors changes in laws and industry standards, automatically updating document checks to reflect new requirements. This consistency helps both buyers and sellers avoid potential legal issues, fostering greater trust and transparency in real estate transactions. Moreover, AI’s ability to reduce manual labor leads to cost savings, making operations more efficient. As a result, AI is becoming a crucial asset in the modern real estate landscape.
The Future of Real Estate: Generative AI and Seamless Property Deals
Generative AI is set to transform the real estate industry by streamlining property transactions. AI-powered platforms can analyze vast data, helping buyers and sellers make quick, informed decisions. Virtual property tours, market trend predictions, and accurate valuations allow investors and agents to strategize more efficiently.
AI also enhances transaction security by automating documentation and reducing fraud. It speeds up the process while delivering personalized recommendations, creating a more seamless, transparent, and efficient property deal experience. The future of real estate, powered by AI, is more efficient and customer-centric than ever.
AI-Driven Data Analytics: Transforming Politics and Securing Elections
Artificial intelligence is transforming the political landscape, driving more sophisticated campaigns and enhancing election security. With the capabilities of AI-driven data analytics, political teams can now sift through massive datasets to identify trends, understand voter behavior, and create targeted messaging that truly resonates with voters. This ability to adapt in real-time ensures that campaigns remain relevant and impactful, tailoring their approaches to the ever-changing political environment.
Beyond shaping campaign strategies, AI also plays a crucial role in safeguarding election integrity. By detecting anomalies in voter data and identifying potential fraud, AI helps maintain the transparency and security of the electoral process. As concerns about election security and transparency continue to grow, AI becomes an indispensable tool in protecting the democratic process and ensuring fair and credible elections.
AI-Enhanced Voter Behavior Insights: Data-Driven Political Decisions
Imagine a world where political messages seem to speak directly to interests and concerns—that’s the transformative power of AI-driven analytics in action. Voters are experiencing a new level of engagement and relevance in political content, thanks to advanced AI tools like Crimson Hexagon and PoliMonitor that analyze vast datasets, including voting history, social media activity, and demographic information. These AI systems offer campaigns deep insights into voter preferences, enabling them to craft highly personalized messages that resonate on an individual level.
This tailored approach means that voters receive content that truly addresses their specific needs and concerns, rather than generic, one-size-fits-all messaging. Additionally, AI’s ability to predict and track changes in voter sentiment in real-time allows campaigns to adjust their strategies dynamically. This responsiveness ensures that political communication remains timely and effective, adapting to shifting attitudes and emerging issues. As AI technology continues to advance, its impact on political messaging is set to deepen, making interactions with campaigns more relevant and engaging, and ensuring that voters are better informed and more connected to the issues that matter most to them.
Detecting and Preventing Election Fraud with AI Algorithms
AI algorithms are revolutionizing the way election fraud is detected, ensuring greater transparency in democratic processes. By analyzing large datasets, AI can swiftly identify irregularities such as duplicate registrations and unusual voting patterns, significantly reducing the potential for human error. Governments are increasingly adopting these technologies to safeguard election integrity, making AI an essential tool in fraud prevention.
Moreover, AI goes beyond mere detection by utilizing predictive analytics to anticipate potential risks and monitoring social media to combat misinformation. As elections become more digitized, AI’s role in maintaining security and fairness is becoming increasingly critical. This underscores the importance of AI for government agencies tasked with election security, as it helps protect against cyber threats and ensures the integrity of election data and processes.
Predictive Analytics: Shaping Campaign Strategies and Election Outcomes
Predictive analytics is transforming campaign strategies and election outcomes by harnessing AI to analyze voter data and trends. By examining information from social media, voting history, and demographics, AI enables campaigns to forecast voter behavior with remarkable accuracy. This capability allows political teams to craft highly targeted messages and strategies tailored to specific voter segments, optimizing their outreach and engagement efforts.
Additionally, AI-powered predictive analytics enhances campaign efficiency by facilitating real-time adjustments. It helps campaigns anticipate shifts in voter sentiment and opponent strategies, enabling better resource allocation and more effective voter engagement. As AI technology continues to advance, its influence on shaping election strategies and outcomes is expected to grow, offering deeper insights and more precise tools for both political analysts and campaign teams.
Social Media Sentiment Analysis: AI’s Role in Political Messaging
AI-driven social media sentiment analysis is transforming political analysis by offering deep insights into voter behavior and election trends. For political analysts, AI tools provide real-time data on public sentiment, enabling more accurate predictions and strategic advice. This enhanced analysis helps campaigns refine their messaging and engage more effectively with voters.
For voters, AI ensures that political content is relevant and engaging, improving the quality of information and interaction. Additionally, government agencies use AI to protect election integrity and combat cyber threats, making elections more secure. As AI technology advances, its impact on political messaging and election security continues to grow.
AI in Election Auditing: Enhancing Transparency and Security
AI in Election Auditing is revolutionizing the way election committees ensure transparency and security. By utilizing advanced AI tools, these committees can efficiently monitor and audit election processes, quickly identifying irregularities and potential fraud. This technology enhances transparency by detecting discrepancies and maintaining trust in the electoral system.
Political analysts also gain from AI, using it to delve deeper into voter behavior, election trends, and public sentiment. AI provides critical insights that improve predictions and strategic advice, leading to more informed and effective election strategies. As AI continues to advance, its impact on both auditing and analyzing elections is becoming increasingly vital for ensuring a secure and transparent democratic process.
Ethical Challenges and the Future of AI in Politics and Election Security
As AI advances in politics and election security, it presents both significant benefits and ethical dilemmas. While AI enhances voter targeting, sentiment analysis, and election integrity, it also raises concerns about privacy, data manipulation, and misuse. These issues underscore the need for strict ethical guidelines to protect voter information and ensure fair practices.
The future of AI in politics will depend on addressing these ethical challenges while leveraging its potential. Ensuring transparency, data protection, and clear regulations will be key to maintaining trust and fairness in elections. Balancing innovation with ethical considerations is essential for upholding democratic values as AI technologies continue to evolve.
Hybrid Cloud Revolution: A Modern IT Infrastructure Solution
Hybrid cloud combines on-premises infrastructure with public cloud services, like AWS, to create a flexible, scalable IT environment. It allows businesses to run workloads locally and in the cloud, optimizing performance, security, and compliance. This architecture offers the agility to manage data where it’s most efficient, balancing the benefits of cloud innovation with the control of in-house systems.
With AWS hybrid cloud solutions such as AWS Outposts and AWS Direct Connect, businesses can seamlessly integrate their existing infrastructure with the cloud. This enables efficient scaling, low-latency connections, and adherence to regulatory standards, providing the best of both on-premises and cloud environments.
Why do businesses implement a hybrid cloud?
Businesses adopt hybrid cloud solutions to integrate their on-premises infrastructure with cloud services like AWS, enhancing control and flexibility. This approach allows for smoother migration and modernization by combining existing IT systems with AWS’s scalability and cost-efficiency. Companies can keep sensitive workloads on-premises while utilizing AWS for its powerful tools and resources, which help meet low-latency needs, process data locally, and comply with regulations.
AWS plays a crucial role in hybrid cloud strategies by offering scalable resources that can adjust to varying demands, reducing hardware investments and operational costs. Additionally, hybrid cloud setups ensure business continuity by enabling workload shifts between on-premises and cloud environments, minimizing disruptions during maintenance or failures. This blend of local and cloud resources helps businesses maintain a resilient and adaptable IT infrastructure.
Key Benefits of Hybrid Cloud for Modern Businesses
Hybrid cloud infrastructure provides modern businesses with a powerful blend of on-premises and cloud solutions, leveraging the best of both worlds to enhance operational efficiency and flexibility. One of the primary advantages of a hybrid cloud is increased scalability. By integrating AWS cloud services with existing on-premises resources, companies can dynamically scale their compute and storage capabilities based on demand. This elasticity ensures that businesses can handle varying workloads efficiently without over-provisioning resources, thereby optimizing costs and performance.
Another significant benefit is improved agility in development and deployment. With a hybrid cloud setup, businesses can leverage AWS’s extensive suite of services to accelerate application development, testing, and deployment. This approach allows for rapid experimentation and innovation, as developers can utilize AWS’s scalable infrastructure to test new ideas and roll out solutions faster than traditional setups. The ability to seamlessly integrate and manage these services across environments further supports continuous integration and delivery practices.
Hybrid cloud solutions also bolster business continuity and resilience. In scenarios where on-premises systems are undergoing maintenance or facing issues, AWS’s public cloud can take over, ensuring minimal disruption to operations. This capability is crucial for maintaining high availability and reducing downtime, which directly impacts customer satisfaction and operational efficiency.
Lastly, hybrid cloud environments enhance compliance and data security. By using AWS’s secure cloud services in conjunction with local data centers, businesses can ensure that sensitive data is stored and managed according to regulatory requirements. This hybrid approach provides greater control over data residency and security policies, helping businesses meet stringent compliance standards while taking advantage of cloud innovation.
Hybrid Cloud with AWS: A Strategic Approach to Modern IT Infrastructure
In today’s rapidly evolving digital landscape, many organizations are turning to hybrid cloud solutions to achieve greater flexibility and scalability. AWS, with its comprehensive suite of cloud services, stands out as a leader in this space. The hybrid cloud strategy offered by AWS allows businesses to seamlessly integrate on-premises infrastructure with AWS’s robust cloud environment. This approach enables organizations to leverage the scalability and cost-efficiency of AWS while maintaining control over sensitive data and legacy systems on-premises. Key AWS services like AWS Direct Connect and AWS Storage Gateway facilitate this seamless integration, ensuring a smooth and secure connection between local data centers and the cloud.
AWS’s hybrid cloud strategy is designed to address the diverse needs of businesses looking to optimize their IT operations. By utilizing services such as Amazon EC2, which provides scalable compute capacity, and Amazon S3, which offers secure and scalable storage, companies can build a hybrid environment that supports a wide range of applications and workloads. This strategic blend of on-premises and cloud resources allows organizations to achieve enhanced performance, agility, and cost-effectiveness. As businesses continue to navigate the complexities of modern IT infrastructure, AWS’s hybrid cloud solutions offer a compelling path forward, combining the best of both worlds to drive innovation and operational excellence.
Building a Hybrid Cloud Strategy for Future Growth
In today’s rapidly evolving technological landscape, adopting a hybrid cloud strategy is becoming increasingly crucial for businesses aiming to scale and innovate. A hybrid cloud environment, which integrates both on-premises infrastructure and public cloud services, offers unparalleled flexibility and agility. By leveraging platforms like AWS, organizations can seamlessly manage workloads across different environments, ensuring optimal performance and cost-efficiency. AWS’s extensive suite of cloud services, including AWS Lambda and Amazon EC2, provides businesses with the tools they need to dynamically allocate resources and adapt to changing demands.
Implementing a hybrid cloud strategy with AWS not only enhances operational efficiency but also fortifies data security and compliance. AWS’s robust security features, such as AWS Shield and AWS Key Management Service (KMS), ensure that sensitive information is safeguarded across both on-premises and cloud environments. Furthermore, the integration of AWS’s cloud-native tools with existing on-premises infrastructure enables organizations to harness the full potential of cloud computing while maintaining control over their data and applications. This strategic approach to cloud adoption empowers businesses to future-proof their IT infrastructure, drive innovation, and support sustained growth in an increasingly competitive market.
Being an AWS Select Partner offers a range of advantages for organizations focusing on hybrid cloud solutions. This partnership provides exclusive access to advanced training and technical support, ensuring that partners are well-equipped to implement and optimize AWS hybrid cloud strategies. AWS Select Partners also benefit from the AWS Partner Network (APN) Portal, which includes specialized tools and resources to enhance their hybrid cloud offerings. Moreover, the partnership opens doors to co-marketing opportunities and collaborative go-to-market initiatives, empowering partners to showcase their expertise and expand their reach in delivering innovative hybrid cloud solutions with AWS.
AI Innovation with AWS Infrastructure and Managed Services
Artificial Intelligence (AI) is transforming industries at an unprecedented pace, and Amazon Web Services (AWS) is at the forefront of this revolution. With its robust infrastructure and comprehensive managed services, AWS empowers businesses to innovate rapidly, scale effortlessly, and achieve their AI goals with precision. From startups to enterprise giants, AWS provides the essential tools and foundational models that fuel AI development, making complex processes more accessible and efficient.
AWS’s AI infrastructure offers a suite of powerful services, including pre-trained foundational models that can be seamlessly integrated into various applications. These models, such as Amazon SageMaker and AWS Lambda, enable businesses to deploy and manage AI workloads without the need for extensive in-house expertise. With AWS’s scalable solutions, organizations can experiment with AI technologies, accelerate model training, and deploy solutions at a global scale—all while maintaining cost efficiency.
In a world where AI capabilities are becoming a key differentiator, leveraging AWS’s infrastructure and managed services positions businesses to stay ahead of the curve, driving innovation and delivering cutting-edge solutions to their customers.
Leveraging AWS Infrastructure for AI Advancements
AWS provides a powerful infrastructure that accelerates artificial intelligence (AI) advancements by offering scalable and high-performance computing resources. With services like Amazon EC2, businesses gain access to flexible computing power that can be tailored to the specific needs of their AI workloads. For more intensive tasks, AWS’s GPU-based instances, such as the P4 and P5 series, deliver the high computational capability required for training complex machine learning models.
Moreover, AWS supports a range of AI tools and frameworks, including TensorFlow, PyTorch, and Apache MXNet, which streamline the development of AI applications. AWS SageMaker further simplifies the process by providing a comprehensive suite of tools for building, training, and deploying machine learning models. With robust data storage solutions like Amazon S3 and Amazon RDS, AWS ensures efficient data management and security, making it easier for businesses to drive AI innovation and achieve technological goals.
Scalable AI Solutions: AWS’s Role in Innovation
AWS is at the forefront of scaling AI solutions, particularly with its robust suite of foundation models. These pre-trained models, available through services like Amazon SageMaker, provide organizations with a powerful starting point for their AI projects. By leveraging these advanced foundation models, businesses can avoid the complexity of building models from scratch and instead focus on customizing and fine-tuning them to meet specific needs. This approach significantly accelerates the development process, allowing for faster deployment and more efficient utilization of resources.
Additionally, AWS’s elastic computing infrastructure ensures that these AI models can scale effortlessly. Whether your application requires intensive training or real-time inference, AWS’s scalable compute resources, including EC2 and SageMaker, can handle varying workloads with ease. This flexibility not only optimizes performance but also reduces operational costs. With AWS’s foundation models and scalable infrastructure, organizations can drive innovation, stay competitive, and rapidly adapt to the evolving demands of the AI landscape.
Streamlining AI Operations with AWS Managed Services
Efficient AI operations hinge on the ability to seamlessly manage complex infrastructures, and AWS Managed Services are pivotal in this process. By offloading routine maintenance tasks and infrastructure management to AWS, businesses can focus on developing and deploying AI models rather than grappling with the intricacies of underlying infrastructure. AWS Managed Services provides automated patching, monitoring, and backups, ensuring that your AI systems run smoothly and securely without requiring constant oversight. This allows data scientists and engineers to devote their expertise to refining algorithms and enhancing model performance.
Furthermore, AWS Managed Services facilitate scalability and flexibility, crucial for AI applications that often experience variable workloads. With on-demand resource scaling, AWS ensures that your AI projects have access to the necessary computational power and storage as needed, optimizing performance and cost-efficiency. By leveraging these managed services, organizations can achieve faster deployment cycles, reduced operational overhead, and improved system reliability, thereby accelerating their AI innovation and gaining a competitive edge in the market.
Optimizing Cloud Management for Enhanced AI Performance
Cloud-native application development is a pivotal approach for optimizing cloud management and boosting AI performance. Unlike traditional applications, cloud-native apps are designed specifically to leverage cloud environments’ inherent flexibility, scalability, and resilience. This method involves building applications as a collection of microservices, which are loosely coupled and can be developed, deployed, and scaled independently. By adopting cloud-native principles, organizations can achieve greater agility, enabling rapid iteration and deployment of AI models and services.
In the context of AI, cloud-native development allows for seamless integration with various cloud-based AI tools and services. For instance, AWS provides a suite of managed services and APIs that can be easily incorporated into cloud-native applications. This integration ensures that AI solutions can scale effortlessly to handle varying workloads and deliver optimal performance. Additionally, cloud-native applications benefit from automated scaling, high availability, and robust disaster recovery, which are crucial for maintaining the performance and reliability of AI-driven applications in a dynamic cloud environment.
Future Trends: AWS and the Evolution of AI Technologies
AWS is at the forefront of AI innovation, driving the future of AI technologies with its robust foundational models. These models, such as Amazon SageMaker and AWS Deep Learning AMIs, provide the building blocks for developing advanced AI applications. By offering pre-trained models and frameworks, AWS enables developers to accelerate their AI projects without starting from scratch. This streamlined approach not only reduces time-to-market but also enhances the accuracy and efficiency of AI solutions.
Looking ahead, AWS’s foundational models are set to play a crucial role in the evolution of AI. The integration of these models with emerging technologies, like quantum computing and advanced data analytics, promises to unlock new possibilities in AI research and application. As AWS continues to innovate, its foundational models will evolve to support increasingly complex and sophisticated AI technologies, driving advancements across various industries and setting new standards for what AI can achieve.
Revolutionizing Logistics with IoT: Tracking, Fleet Management, and Automation
The logistics industry is undergoing a significant transformation, driven by the seamless integration of Internet of Things (IoT) technologies. With its ability to enable real-time tracking, advanced fleet management, and warehouse automation, IoT is revolutionizing the way logistics operations are carried out. By enhancing efficiency, transparency, and operational effectiveness, IoT is reshaping the logistics landscape. In this blog, we will delve into the profound impact of IoT on tracking, fleet management, and automation, and how it is revolutionizing the logistics industry.
Real-Time Tracking: Boosting Supply Chain Transparency
One of the most significant advancements IoT brings to logistics is real-time tracking. Traditionally, monitoring the movement of goods through the supply chain was a challenge fraught with delays and inaccuracies. IoT-enabled GPS devices and sensors have changed that, providing unprecedented visibility into the location and status of shipments.
IoT sensors can track vehicles, shipments, and assets in real-time, delivering precise information about their position and movement. This real-time data helps logistics companies and their clients to gain better transparency across the supply chain. For instance, if a shipment is delayed or rerouted, stakeholders are immediately notified, allowing them to make informed decisions and manage customer expectations.
Additionally, IoT sensors can monitor environmental conditions such as temperature, humidity, and vibration during transit. This is especially crucial for sensitive goods like pharmaceuticals, food, or electronics, where deviations from optimal conditions can lead to spoilage or damage. By setting parameters and receiving alerts if conditions fall outside these ranges, companies can take corrective actions swiftly, ensuring the integrity of their shipments.
Fleet Management: Maximizing Efficiency with IoT
Effective fleet management is critical to the success of logistics operations. IoT technologies offer powerful tools to enhance fleet management by providing real-time data and predictive insights.
Route Optimization: IoT devices collect real-time information on traffic, weather, and road conditions. By analyzing this data, logistics companies can dynamically adjust routes for faster and more efficient deliveries. This not only reduces transit times but also lowers fuel consumption and operational costs. For example, if a traffic jam or severe weather is detected on a planned route, the system can suggest an alternative path, avoiding potential delays.
Predictive Maintenance: Another significant advantage of IoT in fleet management is predictive maintenance. Sensors installed in vehicles monitor performance and detect signs of wear and tear before they lead to breakdowns. By analyzing data on engine health, tire pressure, and other critical components, predictive maintenance systems can schedule repairs and replacements proactively. This approach minimizes unscheduled downtime, enhances safety, and extends the lifespan of vehicles, leading to cost savings and improved operational reliability.
Smart Warehousing: Automating Logistics Operations
Warehousing is a cornerstone of logistics, and IoT is driving a wave of automation and efficiency improvements in this area.
Smart Inventory Management: IoT-enabled sensors and RFID tags offer real-time tracking of inventory levels. This technology automates inventory management processes, such as reordering and stock updates, reducing the risk of stockouts and overstocking. With accurate and timely data on inventory levels, companies can ensure optimal stock levels and avoid costly disruptions in their supply chain.
Automation and Robotics: In modern warehouses, IoT-integrated robots and automated guided vehicles (AGVs) are revolutionizing operations. These systems can perform tasks like picking, sorting, and packing with high precision and speed. Automation reduces the reliance on manual labor, minimizes human errors, and increases overall efficiency. For example, AGVs can transport goods within a warehouse without human intervention, streamlining workflows and optimizing space utilization.
Predictive Maintenance: Reducing Costs and Downtime
Predictive maintenance is a game-changer in logistics, thanks to IoT’s ability to provide actionable insights and early warnings. By continuously monitoring the condition of equipment and vehicles, IoT systems can predict when maintenance is needed before failures occur.
This proactive approach to maintenance reduces the risk of unexpected breakdowns and associated downtime. Instead of waiting for equipment to fail, companies can schedule maintenance activities based on real-time data, ensuring that machinery operates smoothly and reliably. This not only enhances operational efficiency but also leads to significant cost savings by avoiding expensive emergency repairs and extending the lifespan of assets.
The Future of Logistics: IoT’s Growing Influence
As IoT technologies continue to evolve, their influence on the logistics industry will only grow. The integration of advanced analytics, artificial intelligence, and machine learning with IoT data will enable even more sophisticated solutions and insights.
Shortly, we can expect further advancements in predictive analytics, allowing logistics companies to anticipate and respond to potential disruptions with greater accuracy. Enhanced automation and robotics will continue to streamline warehouse operations, while more connected and intelligent fleet management systems will optimize transportation networks.
Moreover, the increasing adoption of IoT in logistics will drive greater collaboration and data sharing among stakeholders, leading to more integrated and efficient supply chains. Companies that embrace these innovations will gain a competitive edge, improving their operational performance and delivering superior service to their customers.
In a nutshell, the integration of IoT technologies into logistics is transforming the industry in profound ways. Real-time tracking, advanced fleet management, and smart warehousing are just a few examples of how IoT is enhancing efficiency, transparency, and operational effectiveness. As these technologies continue to evolve, they will drive further innovations and improvements, shaping the future of logistics and supply chain management. Embracing IoT’s potential will be crucial for companies seeking to stay ahead in a rapidly changing market and deliver exceptional value to their customers.
Fashion Forward: The Generative AI Revolution in E-commerce Retail
As the e-commerce industry evolves, retailers face new challenges and opportunities, making it crucial to integrate Generative AI into their business strategies. This transformative technology is revolutionizing both front-end customer interactions and back-end processes, from personalized search suggestions and AI-generated collections to localized product content. By embracing Generative AI, brands can offer highly personalized shopping experiences, enhance customer engagement, and drive innovation, ensuring they stay competitive in a rapidly changing market. In this blog, we delve into the profound impact of Generative AI on e-commerce, exploring its role in personalized shopping, virtual try-ons, predictive fashion trends, and the future of AI-powered business models.
Personalized Shopping Experiences: AI in eCommerce
Personalization has become the cornerstone of modern eCommerce, and AI is at the forefront of delivering this tailored shopping experience. Generative AI analyzes vast amounts of customer data, including browsing history, past purchases, and even social media behavior, to create individualized recommendations. These personalized suggestions ensure that customers are presented with products that align with their preferences, increasing the likelihood of a purchase.
Beyond product recommendations, AI is enhancing the overall shopping journey. AI-driven chatbots provide real-time assistance, answering customer queries and guiding them through the buying process. These virtual assistants are not just reactive; they proactively suggest products, offer discounts and provide personalized content based on the customer’s behavior. This level of customization not only improves the customer experience but also fosters brand loyalty, as shoppers feel that the brand understands and caters to their unique needs.
Moreover, visual search capabilities powered by AI allow customers to upload images and find similar products, revolutionizing the way people discover new items. This feature is particularly valuable in the fashion industry, where style and aesthetics play a crucial role in purchase decisions. By leveraging AI, eCommerce platforms are creating a more intuitive, engaging, and personalized shopping experience that resonates with today’s consumers.
Virtual Try-Ons: Redefining Customer Engagement
One of the most significant challenges in online fashion retail is the inability for customers to physically try on products before making a purchase. Generative AI is addressing this issue through virtual try-ons, a technology that allows customers to see how clothes, accessories, or makeup would look on them without leaving their homes.
Virtual try-ons use AI to analyze a customer’s body shape, skin tone, and facial features, creating a realistic representation of how products will fit and appear. This technology not only enhances customer confidence in their purchase decisions but also reduces return rates, as customers are more likely to choose items that suit them well.
For example, AI-powered virtual fitting rooms enable shoppers to mix and match outfits, experiment with different styles, and see the results in real time. This interactive experience bridges the gap between online and in-store shopping, offering the convenience of eCommerce with the assurance of a fitting room experience. By redefining customer engagement through virtual try-ons, AI is helping retailers create a more immersive and satisfying shopping experience.
Predictive Fashion Trends: How AI is Shaping the Future
The fashion industry is notoriously fast-paced, with trends emerging and fading at a rapid pace. Generative AI is playing a pivotal role in predicting these trends, enabling retailers to stay ahead of the curve. By analyzing data from various sources, including social media, fashion shows, and consumer behavior, AI can identify emerging trends before they become mainstream.
This predictive capability allows retailers to optimize their inventory, ensuring they stock the right products at the right time. For instance, AI can forecast the popularity of certain styles, colors, or materials, enabling brands to respond quickly to changing consumer preferences. This agility is crucial in the fashion industry, where timing is everything.
Moreover, AI can help designers and brands experiment with new ideas, generating innovative designs based on current trends. These AI-generated designs can inspire fashion lines, allowing brands to create unique collections that resonate with consumers. By leveraging AI’s predictive power, retailers can not only keep up with the latest trends but also set new ones, establishing themselves as industry leaders.
How AI is Revolutionizing the Retail Industry
The impact of AI on the retail industry extends beyond eCommerce and fashion. Across the entire retail landscape, AI is driving innovation, efficiency, and customer satisfaction. From supply chain optimization to in-store experiences, AI is revolutionizing how retailers operate and interact with customers.
In supply chain management, AI is improving efficiency by predicting demand, optimizing inventory levels, and reducing waste. Machine learning algorithms analyze sales data, seasonal trends, and external factors such as economic conditions to forecast demand accurately. This enables retailers to manage their inventory more effectively, ensuring that popular products are always in stock while minimizing excess inventory.
In physical stores, AI is enhancing the shopping experience through technologies such as smart mirrors, automated checkout systems, and personalized promotions. Smart mirrors, for instance, allow customers to try on clothes virtually, offering styling suggestions based on their preferences. Automated checkout systems use AI to streamline the payment process, reducing wait times and improving customer satisfaction.
Moreover, AI-driven personalization extends to in-store promotions, where customers receive tailored offers based on their purchase history and behavior. This level of customization ensures that promotions are relevant, increasing the likelihood of a sale and improving the overall shopping experience.
The Future of Retail: AI-Powered Business Models
As AI continues to evolve, it is paving the way for new business models in the retail industry. AI-powered platforms are enabling retailers to offer highly customized products and services, catering to the specific needs and preferences of individual customers.
One emerging business model is the concept of hyper-personalization, where AI tailors every aspect of the shopping experience to the individual customer. This goes beyond product recommendations and extends to personalized pricing, marketing, and even product design. By leveraging AI, retailers can create unique experiences for each customer, differentiating themselves in a competitive market.
Another promising development is the rise of AI-driven marketplaces, where algorithms match customers with products and services that best meet their needs. These platforms use AI to analyze customer data, predict preferences, and curate personalized shopping experiences. This not only enhances customer satisfaction but also allows smaller brands to reach their target audience more effectively.
Generative AI is transforming the e-commerce retail industry by seamlessly integrating online and offline experiences, ensuring customers receive personalized and consistent interactions across all channels. By tracking online behavior, AI tailors in-store experiences with customized recommendations and promotions, enhancing customer engagement and satisfaction. As AI continues to advance, it is not only driving innovation in personalized shopping and virtual try-ons but also predicting fashion trends and unlocking new business models. Retailers who embrace this technology will be at the forefront of a fashion-forward, customer-centric future in commerce.
Supercharging AWS Cloud Operations: A Journey from Docker to Kubernetes
Understanding the Docker-Kubernetes-AWS Ecosystem
The Docker-Kubernetes-AWS ecosystem forms a robust foundation for modern cloud operations. Docker streamlines the packaging and deployment of applications by containerizing them, ensuring consistent environments across different stages of development. Kubernetes, an orchestration tool, takes this a step further by automating the deployment, scaling, and management of these containers, providing resilience and high availability through features like self-healing and load balancing.
Integrating this with AWS amplifies the ecosystem’s capabilities. AWS offers scalable infrastructure and managed services that complement Kubernetes’ automation, like Amazon EKS (Elastic Kubernetes Service), which simplifies Kubernetes deployment and management. This trio enables organizations to build, deploy, and manage applications more efficiently, leveraging Docker’s portability, Kubernetes’ orchestration, and AWS’s extensive cloud infrastructure. Together, they create a seamless, scalable, and resilient environment that is crucial for cloud-native applications.
Why is Kubernetes the Next Step After Docker on AWS?
After mastering Docker on AWS, Kubernetes emerged as the natural progression for scaling containerized applications. While Docker simplifies container management, Kubernetes takes it further by orchestrating these containers at scale, providing advanced features like automated scaling, self-healing, and seamless rollouts. This level of automation is essential for handling complex workloads in a dynamic cloud environment like AWS, where demands can shift rapidly.
Kubernetes also offers a significant advantage over Docker Swarm in terms of flexibility and resilience. With Kubernetes, you can deploy multi-region, multi-cloud applications effortlessly, ensuring high availability and fault tolerance. Its robust ecosystem, including Helm for package management and integrated CI/CD pipelines, streamlines cloud operations, reducing manual interventions and minimizing downtime. In an AWS environment, Kubernetes leverages services like Amazon EKS, enabling you to manage clusters with AWS-native tools, which enhances security, compliance, and cost efficiency.
In summary, Kubernetes on AWS empowers cloud operations teams with unparalleled control, scalability, and efficiency, making it the logical next step after Docker for enterprises aiming to optimize their cloud-native architectures.
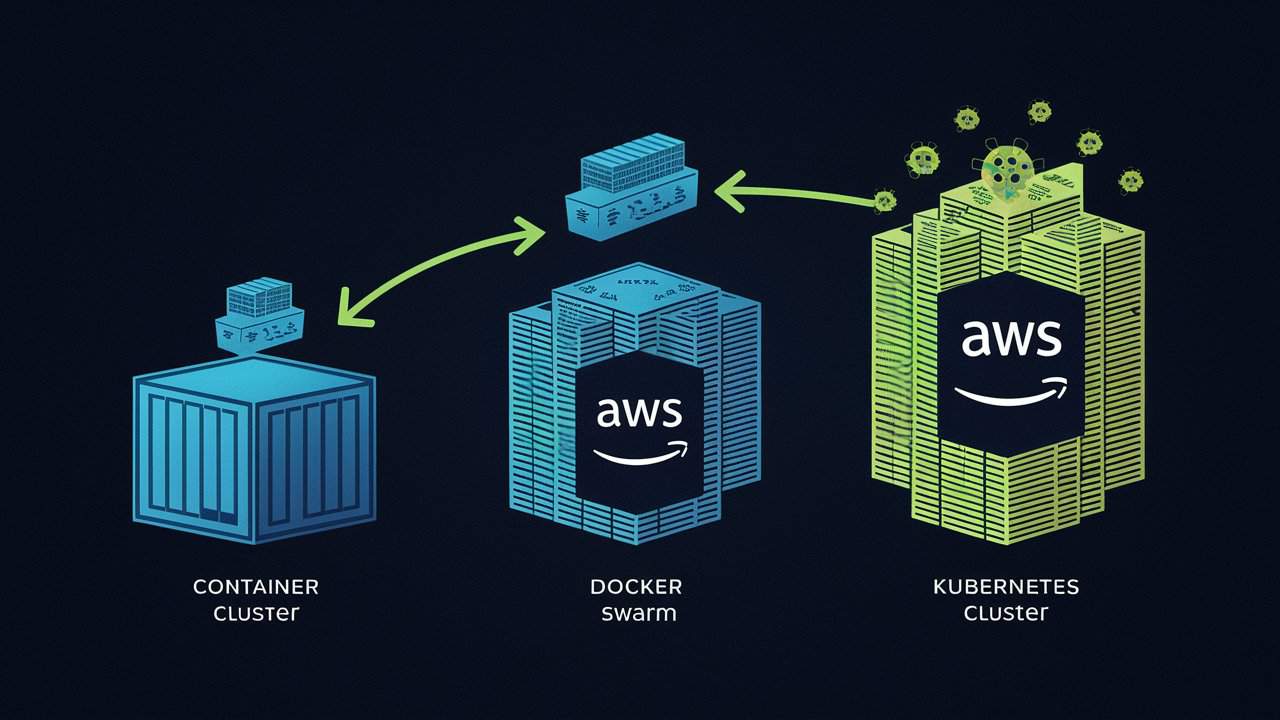
Container Orchestration Simplified: How Kubernetes Enhances Docker Workloads
Container orchestration is pivotal for scaling and managing containerized applications, especially in cloud environments. Kubernetes, an open-source platform, simplifies this by automating the deployment, scaling, and management of Docker containers. It acts as a robust control plane, ensuring that containerized applications run seamlessly across clusters, regardless of underlying infrastructure.
In cloud operations, Kubernetes enhances Docker workloads by providing resilience, scalability, and efficient resource utilization. It automatically handles load balancing, self-healing, and rolling updates, ensuring minimal downtime and consistent application performance. By abstracting the complexity of managing multiple containers, Kubernetes allows cloud teams to focus on application development rather than infrastructure management.
Moreover, Kubernetes integrates smoothly with cloud services like AWS, GCP, and Azure, offering built-in tools for monitoring, logging, and security. This integration ensures that cloud-native applications can scale effortlessly, adapt to changing demands, and maintain high availability, making Kubernetes an indispensable tool for modern cloud operations.
Efficient Application Scaling for Cloud Developers with Docker, Kubernetes, and AWS
Efficient application scaling is vital for cloud developers, and integrating Docker, Kubernetes, and AWS offers a robust solution. Docker streamlines application deployment by encapsulating it in lightweight containers, ensuring consistent performance across different environments. These containers can be easily scaled up or down based on real-time demand, simplifying the management of varying workloads.
Kubernetes enhances this process by orchestrating these containers at scale. As an open-source platform, Kubernetes automates deployment, scaling, and operational management of containerized applications, allowing developers to concentrate on development rather than infrastructure. When paired with AWS, Kubernetes benefits from the cloud provider’s comprehensive ecosystem, including Elastic Kubernetes Service (EKS), which facilitates seamless cluster management.
AWS further supports scalable cloud operations with Auto Scaling and Elastic Load Balancing. These services automatically adjust resources to match traffic fluctuations, maintaining application responsiveness and optimizing cost efficiency. Together, Docker, Kubernetes, and AWS provide a cohesive framework for efficient, scalable cloud management.
Streamlined CI/CD Pipelines: Leveraging Kubernetes in Your Docker-Based AWS Environment
Streamlined CI/CD pipelines are essential for optimizing cloud operations, particularly when integrating Kubernetes with Docker in an AWS environment. Kubernetes automates the deployment, scaling, and management of Docker containers, making it easier to manage complex applications. This orchestration simplifies updates, enhances rollback capabilities, and minimizes downtime, ultimately boosting operational efficiency.
In AWS, combining Kubernetes with Docker leverages the full power of scalable, resilient infrastructure. Services like Amazon EKS (Elastic Kubernetes Service) manage Kubernetes clusters, allowing you to focus on application development rather than infrastructure maintenance. This integration fosters a more agile development process, accelerating time-to-market while ensuring high availability and performance. By aligning your CI/CD practices with these technologies, you achieve a more efficient and reliable cloud operation, meeting the demands of modern software delivery.
Optimizing Docker Swarm and Kubernetes on AWS: Key Takeaways
Optimizing Docker Swarm and Kubernetes on AWS can significantly enhance cloud operations, leading to more efficient resource utilization and streamlined deployments. Docker Swarm’s simplicity is a strong advantage for managing containerized applications. Leveraging AWS features such as Elastic Load Balancing (ELB) and Auto Scaling with Docker Swarm can ensure high availability and dynamic scaling. Utilizing AWS Fargate with Docker Swarm can further optimize operations by removing the need for managing underlying infrastructure, thereby reducing overhead and simplifying management.
On the other hand, Kubernetes provides more advanced orchestration capabilities and is ideal for complex, microservices-based applications. AWS EKS (Elastic Kubernetes Service) integrates seamlessly with Kubernetes, offering managed control plane operations, which simplifies cluster management and scales effortlessly with demand. To optimize Kubernetes on AWS, leverage AWS CloudWatch for monitoring and AWS IAM for fine-grained security controls. Combining Kubernetes’ robust orchestration with AWS’s scalable infrastructure ensures resilient, cost-effective, and highly available cloud operations.